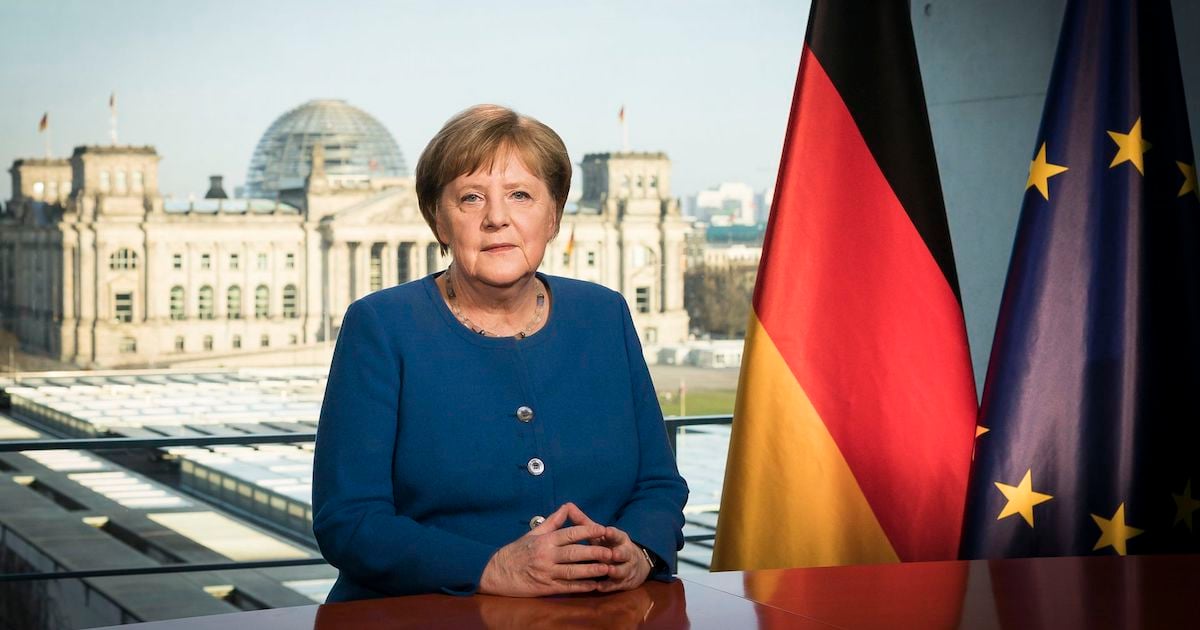
Nowcasting – Germany’s realistic R calculation for COVID-19
The Coronavirus is still dominating both our lives and the news. Every day we hear about numbers of total infections, new infections, and in this context also about the reproduction rate R. Most people might now have a rough understanding about R, and that we need to get it smaller than 1 in order to get rid of the virus. Often we hear about R and its current value, but how current is it really? Inevitably there are delays in reporting, since it takes time from testing a person, to getting the result, to reporting this result, to publishing it. Also, it takes some time from getting infected to developing symptoms, as well as there are undiscovered or simply unreported cases, which all makes it nearly impossible to get accurate numbers, especially numbers close to real time.
With this delay in mind, it is obvious that the numbers we currently have might not be immediate enough for e.g. political decisions, since those decisions are likely to be made a little too late. Also, whenever something changes in the behavior of the public, it is only being reflected in numbers after a certain amount of time, which again leads to possibly wrong perceptions about the situation.
Even though this situation is difficult, thanks to a lot of data already available and mathematics, it is possible to get a quite accurate "guess" of actual recent numbers.
About R
Just a small reminder about what R is and how to think about it. I will not use a detailed mathematic description but instead try to give an intuition for why it is important to keep it low. First thing to mention is, that there are different "types" of R that can easily be confused.
Difference between R0 and Rt
R0
This is the basic reproduction number with "0" meaning generation 0, i.e. in a population that was not yet exposed to a new disease. Thus it states how many individuals likely will get infected by someone in a given population of non-immune individuals. It is being estimated from mathematical models based on real available data.
R0 depends on factors like density and mobility in a given population, meaning that it is likely spreading faster in places with many people who interact and maybe travel a lot, than in places with relatively few interactions between people. By the way, this is why it is important to stay at home and keep distance to others when being outside. It simply helps preventing the virus to spread.
Because of these factors, the value for the same disease can differ from population to population.
Since this basic reproduction number is not time-dependent, it doesn't give a lot of information about how fast a disease is spreading, though, only how many people likely get infected. Here another R comes into play.
Rt
The time-dependent case reproduction number (or effective reproduction number) states how many individuals get infected at a given time. Obviously this number is being subject to change over time, depending on measures like vaccine or rising immunity in the population after a certain amount of individuals being exposed to the disease.
It is being calculated based on R0, but effectively delivers the average number of people an infected person passes the virus to, considering a certain percentage of immunity in a population and countermeasures like a lockdown, for instance. Of course, factors like immunity are changing with time, and so is Rt.
This is the R that is dominating the news in times of COVID-19. Even small differences in R can make a huge difference in the development and speed of spreading. This is due to exponential growth. In order to eventually get rid of the coronavirus, we know that we must keep it smaller than 1. Let's see why.
Exponential growth
A very schematic figure of exponential growth can be seen below, feel free to play around and adjust the slider to get a feeling of what it means to grow exponentially. You can zoom in and out to see changes on a large/small scale as well.
Please note that this simulation is very very simple and schematic. To actually model the Coronavirus numbers, a lot more modeling and statistic calculations is necessary. This is only for getting the very basic idea behind exponential growth!
But even with this basic simulation, the change in the graph when r=1 is obvious. It turns into a straight line, which, in terms of disease spreading, means that one infected person passes the virus to only one other individual.
Due to this very nature of exponential growth, small changes can make a huge difference, especially those changes that are close to R=1, since this is the crucial turning point: growing or declining. For this reason, it is important to not only rely on instincts and "felt" reality, but to monitor the development of R carefully. Like stated above, inevitably there are delays in reporting, so statistical calculations of the given data makes it possible to correct them to a more accurate, recent reflection of the situation.
What is Nowcasting?
Nowcasting is roughly said "guessing" the number of actual new infections at a given time based on real data of reported cases. The goal is to get statistics about when individuals actually got infected with the coronavirus, and not when these cases got reported.
Of course it is not simply guessing. Nowcasting, just as forecasting, is performed with given real data and statistical means applied to it. Nowcasting is being used in other fields like economy or meteorology, but now Germany's Robert Koch Institut (RKI), which is the main and official source for the numbers of infections in Germany, started using this method in their research about Covid-19 as well.
Nowcasting works with calculating data in a sliding time interval of 4 days. At the beginning, data 4 consecutive days about reported infections is used for calculating the first value of R. Of course there is no value until 4 days after the very first report. But once this first value is given, it is used for calculating further values, based on the data of – again – 4 days ago until today. This process of calculations is being repeated, depending on both reported data and the priorly obtained value of R. As a consequence of this technique, nowcasting data is only being published for up to 4 days ago, since more recent data is statistically not reliable enough yet. It needs to be noticed that those number do not reflect how many people actually got infected that day (these likely happened 8-13 days prior), but a guessing about new infections that are likely to happen on this day.
Recently (May 14th, 2020), Germany's RKI started publishing a second nowcasting number which is being calculated for a sliding interval of 7 days. This number is more stable since it is less affected by sudden changes, but it is also a few days less up to date. Nevertheless, the combination of both nowcasting figures is giving a good picture about the current situation.
Summary
In times like this, when accurate recent numbers are needed for important decisions that effect millions of people, the power of mathematics and statistics is a gift. Thanks to many hospitals, research institutions and other sources, there is a lot of data available about COVID-19 infections, but raw data alone is quite meaningless if no evaluation is applied to it. Nowcasting is a great way to make precise guesses about close-to-realtime developments of the situation, based on already evaluated data.
By having this sort of close-to-realtime data available, we can work on keeping that infamous R below 1.