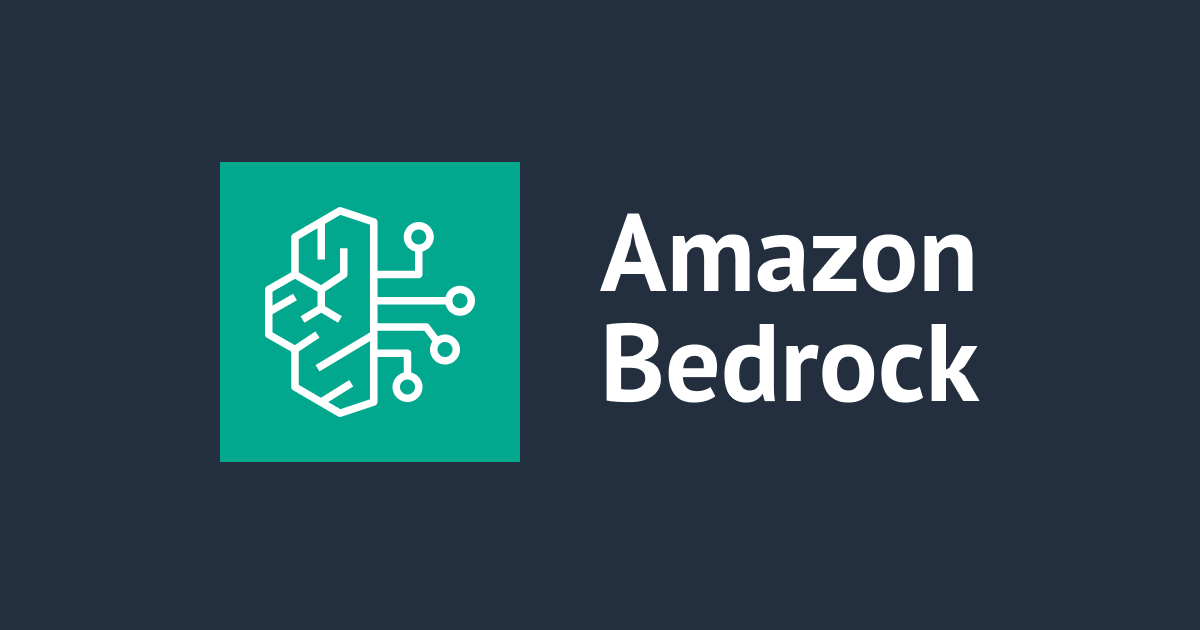
Bedrockでアイキャッチ画像1000個の説明文を作成してみた(Claude 3 Haiku)
当ブログサイト(developersio)、執筆者が指定したアイキャッチ画像が、記事本文の冒頭や一覧表示に反映される仕様となっています。
執筆者がアイキャッチ画像を指定する際、画像のファイル名が短縮名であるなど表記揺れある場合や、 適切なカテゴリ分類から外れていると目的の画像まで到達しにくい課題がありました。
そこで今回、2024年5月時点で約47,000件の技術記事より、複数回の利用実績がある1000個のアイキャッチ画像ファイルを選抜。 Bedrockを活用してClaude 3の画像処理を行い、得られた説明文をCMSの画像検索対象とすることで、アイキャッチ画像の検索性向上を図る機会がありましたので、紹介させて頂きます。
モデルの評価
Amazon Bedrock の チャットのプレイグラウンドを利用して、 Claude 3 の Haiku、Sonnet、Opus のそれぞれのモデルの結果を比較しました。
文字数を制限、短い説明を求めた場合、 Haiku が十分な精度で利用できる事を確認、 レイテンシーが最も少なく、低コストで利用できる Haikuを採用しました。
- プロンプト
この画像について、日本語で400文字以内で、一行で説明してください。
シンプルアイコン
AWSシンプルアイコン (SecurityHub)
- Haiku 応答内容
この画像は AWS (Amazon Web Services) のセキュリティ関連のサービスを示すものです。赤い四角形の中には AWS のセキュリティ製品のロゴが描かれており、右側に「AWS Security Hub」と大きく表示されています。AWS Security Hub は、AWS 環境全体のセキュリティ状況を一元的に可視化・管理するサービスで、セキュリティ上の課題を迅速に特定し、対策を講じることができます。この画像は、AWS の包括的なセキュリティソリューションを象徴するものと言えます。
複数要素デザイン
- Haiku 応答内容
この画像は、Amazon Web Services(AWS)の製品やサービスを示しています。AWS は、クラウド コンピューティングのトッププロバイダーであり、IaaS、PaaS、SaaS を提供しています。この画像は、AWS が提供する様々なサービス(Amazon Redshift、Amazon S3、Amazon EC2、Amazon VPC、Amazon DynamoDB、Amazon CloudWatch など)をアイコン化して表しており、AWS の広範なクラウドソリューションを包括的に示しています。
所要時間
Claude 3 Haiku | Claude 3 Sonnet | Claude 3 Opus |
---|---|---|
2329 ms | 3325 ms | 14364 ms |
2750 ms | 2878 ms | 7927 ms |
実装
Boto3を利用したPythonスクリプトのサンプルを元に、画像処理を追加しました。
Bedrock
エンコード済みの画像データと、media_typeを引数で受け取る変数を用意しました。
import boto3 import json def get_image_description(image_data, image_media_type): request_body = { "anthropic_version": "bedrock-2023-05-31", "max_tokens": 2000, "messages": [ { "role": "user", "content": [ { "type": "image", "source": { "type": "base64", "media_type": image_media_type, "data": image_data, }, }, { "type": "text", "text": "この画像に文字列が含まれている場合、その文字列を最初に表示してください。次にこの画像について、日本語で400文字以内で、一行で説明してください。" } ] } ] } model_id = 'anthropic.claude-3-haiku-20240307-v1:0' accept = 'application/json' content_type = 'application/json' bedrock_runtime = boto3.client("bedrock-runtime", region_name="us-west-2") response = bedrock_runtime.invoke_model( body=json.dumps(request_body), modelId=model_id, accept=accept, contentType=content_type ) response_body = json.loads(response.get('body').read()) return response_body["content"][0]["text"]
PIL
指定したURLから画像をダウンロード。
PIL(Python Image Library)を利用して、解像度、MimeTypeなどの画像情報を取得しました。
from PIL import Image from io import BytesIO import requests import os def get_image_info(url): # 画像をダウンロード response = requests.get(url) response.raise_for_status() image = Image.open(BytesIO(response.content)) # 解像度、MimeType、ファイルサイズを取得 geometry = f"{image.width}x{image.height}" mimetype = response.headers.get('Content-Type', 'application/octet-stream') imageSize = len(response.content) file_name = os.path.basename(url) title = os.path.splitext(file_name)[0] return { 'url': url, 'file': file_name, 'geometry': geometry, 'mimetype': mimetype, 'imageSize': imageSize, 'title': title, }
処理定義
以下の処理を定義しました。
- 指定URLの画像データをダウンロードし、MIMEエンコード
- Bedrockを利用して画像解析
- 画像解析結果、改行区切りのJSONで出力
def process_image(url): image_data = base64.b64encode(requests.get(url).content).decode("utf-8") image_description = get_image_description(image_data, image_info['mimetype']) image_info['description'] = image_description with open('./out.txt', 'a') as e: print(json.dumps(image_info, ensure_ascii=False), file=e)
所要時間、コスト
1000個の画像解析、1並列で実行した所要時間は45分でした。
Bedrockのオンデマンド課金対象となるトークン数より求めた、Haikuモデルの課金額は0.83ドル。
対象 | 数量 | 課金額(USD) |
---|---|---|
InputTokenCount | 1974110 | 0.49 |
OutputTokenCount | 272198 | 0.34 |
合計 | 1747 | 0.83 |
画像検索用の手がかりとなるテキスト、1画像あたり0.1円程度で用意する事ができました。
効果
画像解析で求めた説明文を対象にした全文検索により、関連するアイキャッチ画像の絞り込みが可能になりました。
補足
利用したスクリプトの全文です。
Bedrockのフルアクセス権限が可能なIAMロールを付与した Amazon Linux 2023 のEC2環境を用意。 以下パッケージとモジュールを追加インストールして実行しました。
sudo dnf install pip -y pip install Image boto3
import os import requests from PIL import Image from io import BytesIO import base64 import boto3 import json def get_image_info(url): """ 指定されたURLの画像の情報を取得する Args: url (str): 画像のURL Returns: dict: 画像の情報を格納したディクショナリ - url: 画像のURL - file: ダウンロードした画像のファイル名 - geometry: 画像の解像度 - mimetype: 画像のMimeType - imageSize: ファイルサイズ (バイト) - title: ファイル名から取得したタイトル """ try: # 画像をダウンロード response = requests.get(url) response.raise_for_status() image = Image.open(BytesIO(response.content)) # 解像度、MimeType、ファイルサイズを取得 geometry = f"{image.width}x{image.height}" mimetype = response.headers.get('Content-Type', 'application/octet-stream') imageSize = len(response.content) file_name = os.path.basename(url) title = os.path.splitext(file_name)[0] return { 'url': url, 'file': file_name, 'geometry': geometry, 'mimetype': mimetype, 'imageSize': imageSize, 'title': title, } except (requests.exceptions.RequestException, IOError) as e: print(f"Error getting image info: {e}") return None def get_image_description(image_data, image_media_type): """ Bedrock APIを使って、画像の説明を生成する Args: image_data (str): 画像のBase64エンコードデータ image_media_type (str): 画像のMimeType Returns: str: 生成された説明 """ request_body = { "anthropic_version": "bedrock-2023-05-31", "max_tokens": 2000, "messages": [ { "role": "user", "content": [ { "type": "image", "source": { "type": "base64", "media_type": image_media_type, "data": image_data, }, }, { "type": "text", "text": "この画像に文字列が含まれている場合、その文字列を最初に表示してください。次にこの画像について、日本語で400文字以内で、一行で説明してください。" } ] } ] } model_id = 'anthropic.claude-3-haiku-20240307-v1:0' accept = 'application/json' content_type = 'application/json' bedrock_runtime = boto3.client("bedrock-runtime", region_name="us-west-2") response = bedrock_runtime.invoke_model( body=json.dumps(request_body), modelId=model_id, accept=accept, contentType=content_type ) response_body = json.loads(response.get('body').read()) return response_body["content"][0]["text"] def process_image(url): """ 指定されたURLの画像を処理し、説明を生成してJSON出力する Args: url (str): 画像のURL """ image_info = get_image_info(url) if image_info: print(f"URL: {url}, Geometry: {image_info['geometry']}") if image_info['geometry'] == '1200x630': image_data = base64.b64encode(requests.get(url).content).decode("utf-8") image_description = get_image_description(image_data, image_info['mimetype']) image_info['description'] = image_description with open('./out.txt', 'a') as e: print(json.dumps(image_info, ensure_ascii=False), file=e) else: print(f"Failed to get image info: {url}") with open('./in.txt') as f: for line in f: process_image(line.strip())