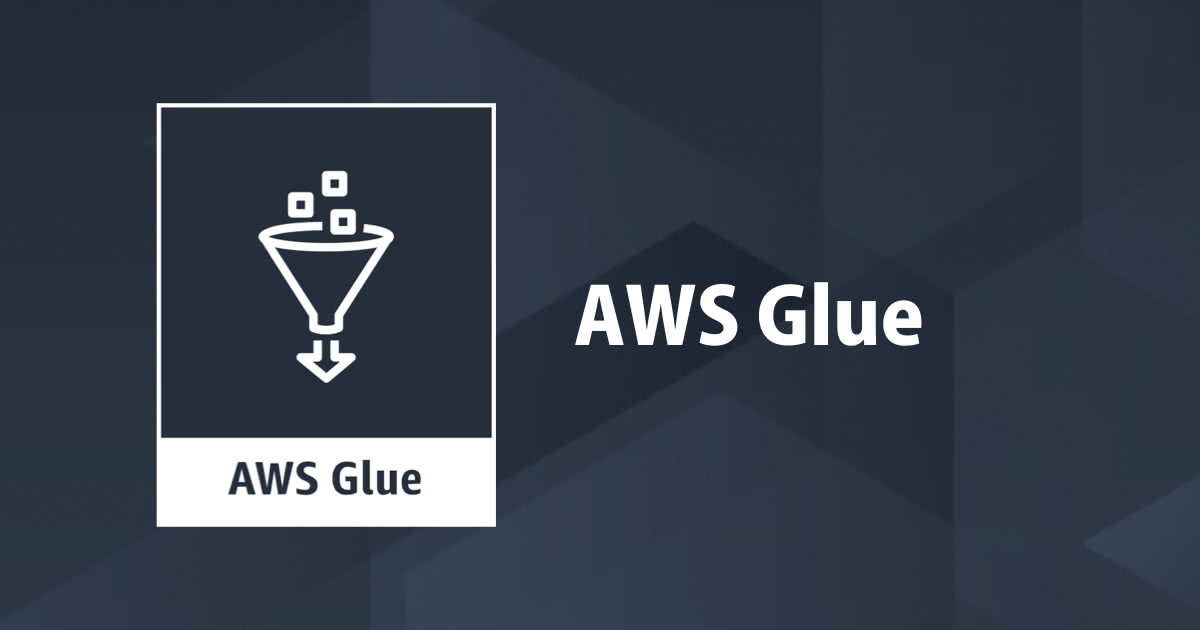
AWS Glueでカンマ区切りの数字(String型)を数値(Long型など)に変換したい
下記のように、カンマ区切りで入力されている数字を数値に変換しようとしていました。
Total Credits Purchased,Total Credits Used,Total Complimentary Credits,Remaining Customer Experience Credits "9,591","6,845",0,0 "30,000","22,498",0,0
Glueだと、DynamicFrameのApplyMappingを使って型の変更ができるので、真似をしてやってみたところ、数値に変換されずにnullになってしまいました。
変換元のCSVのデータ
+---------------------+----------------+-------------------------+----------------------------------+ |totalcreditspurchased|totalcreditsused|totalcomplimentarycredits|remainingcustomerexperiencecredits| +---------------------+----------------+-------------------------+----------------------------------+ | 9,591| 6,845| 0| 0| | 30,000| 22,498| 0| 0| +---------------------+----------------+-------------------------+----------------------------------+
このデータを変換しようと
applymapping1 = ApplyMapping.apply(frame = datasource0, mappings = [ ("totalcreditspurchased", "string", "total_credits_purchased", "long"), ("totalcreditsused", "string", "total_credits_used", "long"), ("totalcomplimentarycredits", "long", "total_complimentary_credits", "long"), ("remainingcustomerexperiencecredits", "long", "remaining_customer_experience_credits", "long") ], transformation_ctx = "applymapping1") applymapping1.toDF().show()
DynamicframeのApplyMapping
を使って変換する方法を使ってみたところ、
+-----------------------+------------------+---------------------------+-------------------------------------+ |total_credits_purchased|total_credits_used|total_complimentary_credits|remaining_customer_experience_credits| +-----------------------+------------------+---------------------------+-------------------------------------+ | null| null| 0| 0| | null| null| 0| 0| +-----------------------+------------------+---------------------------+-------------------------------------+---------+
string形式のデータがnullに変換されてしまいました。
pythonでもカンマ区切りの数字を数値に変換できなかったです。
上記のようにカンマを取り払った後に数値に変換できました。
ということは同じようにpysparkでもカンマを取り払ってあげればできるのでは? と思い色々と調べたところ、
sparkのDataframeのwithColumn
を使用すればできそうということがわかりました。
DataframeにwithColumnは新しいカラムを追加したり、新しい値で置換することができます。
data_df = datasource0.toDF() data_df = data_df.withColumn('totalcreditspurchased', translate( "totalcreditspurchased", ",", "" )) data_df.show()
元のデータソースをDynamicframeからSparkのDataframeに変換してからwithColumn
を使って既存のカラムの値を置き換えしてみたところ、
※ translate()
関数を使ってDataFrameのカラムの値を置き換えました
Dynamicframeで同じようなことができるかどうかは調べきれていません。
+---------------------+----------------+-------------------------+----------------------------------+ |totalcreditspurchased|totalcreditsused|totalcomplimentarycredits|remainingcustomerexperiencecredits| +---------------------+----------------+-------------------------+----------------------------------+ | 9591| 6918| 0| 0| | 30000| 22531| 0| 0| +---------------------+----------------+-------------------------+----------------------------------+
このようにカンマを除外してDataframeに格納できました。やったぜ。
最後はDataframeをDynamicframeに戻して、もともとGlueのJOBで使用していたApplyMapping
で型を変換してs3に書き出すことができました。
## DataframeをDynamicframeに戻す datasource_cast = DynamicFrame.fromDF(data_df, glueContext, 'datasource_cast') ## 元々やりたかったこと applymapping1 = ApplyMapping.apply(frame = datasource_cast, mappings = [ ("totalcreditspurchased", "string", "total_credits_purchased", "long"), ("totalcreditsused", "string", "total_credits_used", "long"), ("totalcomplimentarycredits", "long", "total_complimentary_credits", "long"), ("remainingcustomerexperiencecredits", "long", "remaining_customer_experience_credits", "long") ], transformation_ctx = "applymapping1") 〜〜〜〜〜〜 〜〜〜〜〜〜 〜〜〜〜〜〜 ## s3に書き出し datasink4 = glueContext.write_dynamic_frame.from_options(frame = dropnullfields3, connection_type = "s3", connection_options = {"path": output_dir}, format = "parquet", transformation_ctx = "datasink4") job.commit()
最終的に書き出ししたいDynamicframeのスキーマを出力すると、以下のような定義になっていました。
StructType([Field(total_credits_purchased, LongType({}), {}),Field(total_credits_used, LongType({}), {}),Field(total_complimentary_credits, LongType({}), {}),Field(remaining_customer_experience_credits, LongType({}), {})], {})
ちゃんとLongTypeに変更されていて一安心。