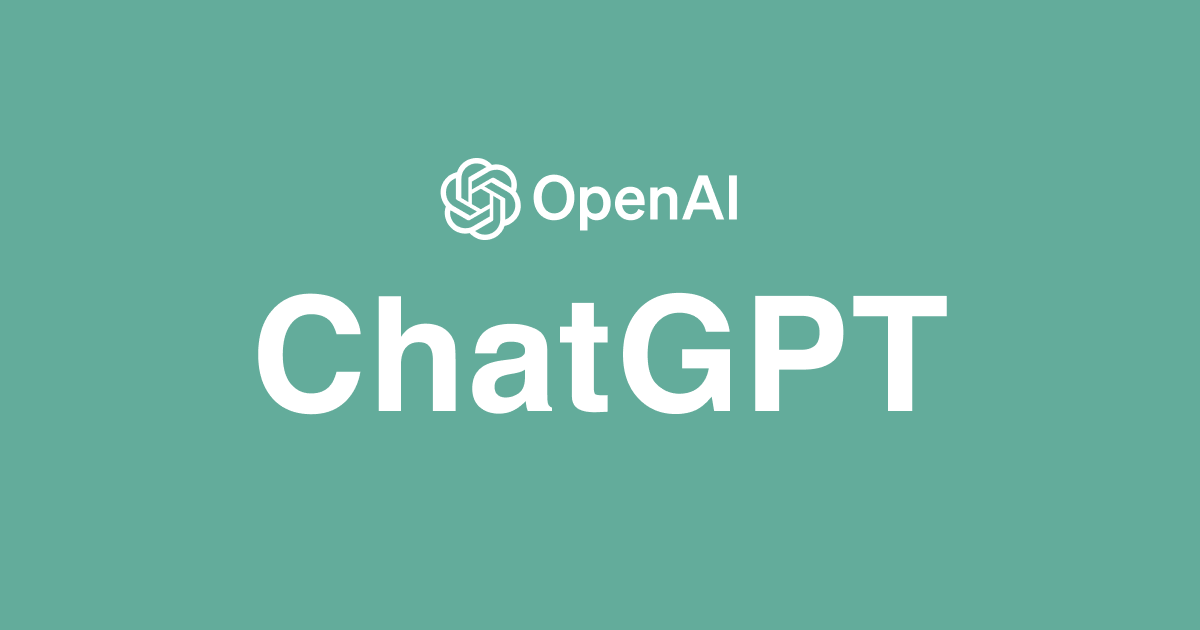
ChatGPT3で箇条書きメモからレポート文を出力して何となく見えてきたNotionAIとChatGPTそれぞれの活用スタイル #ChatGPT #NotionAI
先日箇条書きメモを元にNotionAIでレポート作成を試みる記事を書きましたが、別のAIでの結果を試してみることにしました。ChatGPTによる出力です。とりあえず試してみるという点にて今回はChatGPT3にしています。
ChatGPT3による出力
指示は以下の通り。該当の箇条書きは元記事より持ってきたものです。
以下の箇条書きを元に段落毎に見出しもつけた英語でのレポートを作成し、日本語訳にて書き出して。
問題はトークン数ですが、日本語訳は文字数の問題で英語出力と分けても全ては収まりませんでした。以下、英文と出力された範囲での日本語訳を掲載します。
Data-Centric Approach and Amazon SageMaker
Introduction
Machine learning (ML) is a powerful tool that allows us to build predictive models using large amounts of data. However, traditional approaches to machine learning, such as the model-centric approach, do not always consider the quality of the data being used. In contrast, the data-centric approach focuses on improving the quality of the data, which can lead to significant improvements in model accuracy. In this report, we will explore the benefits of the data-centric approach and how Amazon SageMaker can help data scientists implement it.Benefits of the Data-Centric Approach
The data-centric approach involves cleaning and improving the quality of the data, such as by formatting and addressing missing values. Research has shown that there can be up to a 10% difference in accuracy between clean and dirty data. The machine learning workflow includes three steps: data processing, model development, and deployment. Data processing involves data collection, labeling, exploration, and feature engineering. Model development includes preprocessing, model selection, training, tuning, and evaluation. Deployment involves deploying the model. However, the reasons why machine learning projects fail are due to insufficient data quality and the absence of specialized professionals such as data scientists. To build a successful machine learning project, it is important to have knowledge of machine learning, the ability to quickly try and improve, and a focus on differentiation.Data Processing and Model Development
Automated machine learning (AutoML) technologies such as SageMaker can help automate and standardize the process from model development to deployment. SageMaker Data Wrangler provides a quick and easy way to prepare data for machine learning by improving data quality and exploration, as well as enriching data. SageMaker Autopilot automatically creates machine learning models with complete visibility into the model development process. It also automatically selects the type of prediction and can be linked with SageMaker Data Wrangler for model deployment. SageMaker Canvas generates accurate predictions without requiring the generation of prediction code.Unstructured Data Pattern and Amazon SageMaker
SageMaker Ground Truth creates high-quality datasets for machine learning, particularly for unstructured data such as images, documents, and speech. SageMaker JumpStart offers pre-built solutions to common machine learning problems.Conclusion
The data-centric approach offers a significant advantage over the model-centric approach, which does not consider the quality of the data used. By focusing on data quality, the accuracy of machine learning models can be significantly improved. Amazon SageMaker provides a suite of tools to help data scientists automate and standardize the machine learning workflow, from data processing to model development and deployment.
断片ながら出力された日本語訳は以下。
はじめに
機械学習(ML)は、大量のデータを使用して予測モデルを構築することを可能にする強力なツールです。ただし、モデル中心アプローチなどの従来の機械学習手法では、使用されるデータの品質を常に考慮しているわけではありません。対照的に、データ中心アプローチは、データの品質を改善することに焦点を当てており、これによりモデルの精度が大幅に向上することがあります。このレポートでは、データ中心アプローチの利点と、Amazon SageMakerがデータサイエンティストが実装するのを助ける方法について探究します。データ中心アプローチの利点
データ中心アプローチには、データのクリーニングと品質の改善(例:フォーマット変換や欠損値の対処)が含まれます。クリーンなデータと汚れたデータの間には、精度に10%の差があることが研究によって示されています。機械学習のワークフローには、データ処理、モデル開発、およびデプロイメントの3つのステップがあります。データ処理には、データ収集、ラベリング、探索、および特徴量エンジニアリングが含まれます。モデル開発には、前処理、モデル選択、トレーニング、チューニング、および評価が含まれます。デプロイメントには、モデルのデプロイメントが含まれます。しかし、機械学習プロジェクトが失敗する原因は、データ品質が不十分であることや、データサイエンティストなどの専門家が不在であることです。成功した機械学習プロジェクトを構築するには、機械学習の知識、素早い試行と改善の能力、および差別化に焦点を当てることが重要です。
わかったことと改善点
全体を通して言えることは、箇条書きの内容自体は違えていません。NotionAIとの出力で大きく異なるのが分かる点は、ChatGPTは全体を通して汲み取った後にキーワードとなるフレーズを元に文章を構成しています。NotionAIは箇条書きの順序を優先しているため、SageMakerに関する記載は最後の段落まで出てきません。
ChatGPT3の限界という可能性を考慮する必要はもちろんありますが、見出しとテキスト内容がマッチしているとは言い切れません。この辺りはChatGPT4の活用にて改善する見込みがあります。
あとがき
日本語訳は実際にだされた部分的な出力を持ってきましたが、内容の確認時にはChatGPT上で出力された英訳をDeepLの翻訳用拡張機能を通して段落毎に見ました。
出力したいレポート構成次第ですが、元のメモの記載順に沿った内容としたい場合にはNotionAIを、全体を俯瞰した上でのレポート生成を行いたい場合にはChatGPTを利用するとよいかもしれません。
今回のChatGPT用プロンプトも正直こなれているものとは言い難く、より細かい指示を出すことで構成を誘導することも可能でしょう。