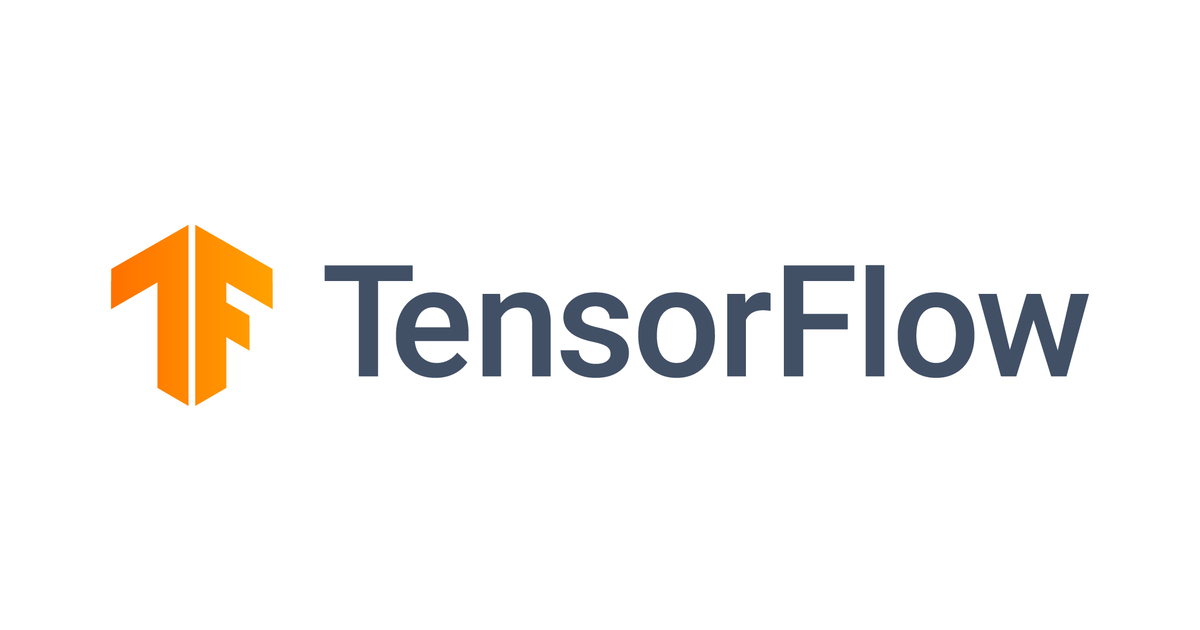
Keras(TensorFlow)のImageDataGeneratorをカスタマイズする
こんちには。
データアナリティクス事業本部機械学習チームの中村です。
今回は、画像データの水増し(Data Augmentation)が可能な、KerasのImageDataGeneratorのカスタマイズ方法について紹介します。
ImageDataGeneratorの基本的な使い方は、以下の前回記事を参照ください。
カスタマイズの概要
カスタマイズの概要は以下です。
- ImageDataGeneratorを継承するクラスを作成する。
- flowという関数を修正する。
- whileループ内でyieldで返す関数として実装する。
- whileループ内ではカスタムでいれたい変換処理を実装する。
今回はこの方法でrandom erasingという手法を導入してみます。
random erasingについて
random erasingは画像の一部をマスクすることで、特定部位の特徴に依存しないようにする手法です。
オクルージョン自動で生成し、汎化性能を上げる工夫という風にも捉えられます。
パラメータとしては、以下を準備する必要があります。
- random erasingを発生させる確率
- マスクする面積の範囲(相対値で表現)
- アスペクト比の範囲
詳細は以下の元論文を参照ください。
変換の実装
前準備
モジュールのインポートやデータ取得を行います。
import tensorflow as tf import tensorflow.keras as keras import numpy as np import matplotlib.pyplot as plt
前回と同じく、データはCIFAR-10というRGB画像のデータセットを使います。
dataset = keras.datasets.cifar10 (train_images, train_labels), (test_images, test_labels) = dataset.load_data()
前回同様、一つの画像を複製します。
sample_index = 7 train_image_sample = train_images[sample_index] train_label_sample = train_labels[sample_index]
この画像を描画しておきます。(いつものお馬さんですね)
plt.figure(figsize=(3,3)) plt.imshow(train_sample) plt.tick_params(labelbottom='off') plt.tick_params(labelleft='off')
カスタムクラス作成
以下のようにImageDataGenerator
を継承したカスタムクラスを作成します。
class MyImageDataGenerator(keras.preprocessing.image.ImageDataGenerator): def __init__(self, random_erasing_probability = None, random_erasing_area_ratio = [0.02, 0.4], random_erasing_aspect_ratio = [0.3, 1/0.3], random_erasing_mask_value = [0, 1], *args, **kwargs ): super().__init__(*args, **kwargs) self.random_erasing_probability = random_erasing_probability self.random_erasing_area_ratio = random_erasing_area_ratio self.random_erasing_aspect_ratio = random_erasing_aspect_ratio self.random_erasing_mask_value = random_erasing_mask_value def random_erasing(self, X, erasing_probability, erasing_area_ratio_range, erasing_aspect_ratio_range, random_erasing_mask_value ): # ...一旦省略... def flow(self, seed=None, *args, **kwargs): batch_gen = super().flow(seed=seed, *args, **kwargs) while True: batch_x, batch_y = next(batch_gen) # random erasing if self.random_erasing_probability is not None: batch_x = self.random_erasing( batch_x, self.random_erasing_probability, self.random_erasing_area_ratio, self.random_erasing_aspect_ratio, self.random_erasing_mask_value) yield (batch_x, batch_y)
__init__
でカスタム処理で使うパラメータを引数に追加します。各パラメータは以下の通りです。
random_erasing_probability
: random erasingを発生させる確率random_erasing_area_ratio
: マスクする面積の範囲(相対値で表現)random_erasing_aspect_ratio
: アスペクト比の範囲random_erasing_mask_value
: マスクする値の取りうる範囲
random_erasing_mask_value
は、元論文に記載がありませんが、画像の画素値の正規化を
どこで行うかによって、設定を変更する場合が都合が良い場合があるため、準備しています。
flow
の部分も以下のように変更しています。
- 継承元の
flow
を実行してgeneratorを取得します。 - whileループ内では、まずgeneratorからバッチデータを取得します。
- そして入力データ
batch_x
をrandom_erasing関数で処理したものに上書きし、ラベルbatch_y
とともにyieldで返します。
random erasing処理は別途関数として以下のように定義します。
(関数化しておいた方が複数のデータ拡張をカスタマイズする時に全体の見通しがよさそうです。)
def random_erasing(self, X, erasing_probability, erasing_area_ratio_range, erasing_aspect_ratio_range, random_erasing_mask_value ): X_copy = X.copy() batch_size, H, W, C = X_copy.shape original_area = H * W for batch_index in range(batch_size): if erasing_probability < np.random.rand(): continue # はみ出さないようリトライするループ while True: # マスクする面積をサンプリング erasing_area = np.random.uniform( erasing_area_ratio_range[0], erasing_area_ratio_range[1] ) * original_area # マスクするアスペクト比をサンプリング erasing_aspect_ratio = np.random.uniform( erasing_aspect_ratio_range[0], erasing_aspect_ratio_range[1] ) # 面積とアスペクト比から高さと幅を計算 erasing_height = int(np.sqrt(erasing_area * erasing_aspect_ratio)) erasing_width = int(np.sqrt(erasing_area / erasing_aspect_ratio)) # マスクを配置する端点をサンプリング erasing_left_top_x = np.random.randint(0, W) erasing_left_top_y = np.random.randint(0, H) # マスクが元画像をはみ出すかどうかを計算 if erasing_left_top_x + erasing_width <= W \ and erasing_left_top_y + erasing_height <= H: break # マスクする値の生成 erasing_values = np.random.uniform( random_erasing_mask_value[0], random_erasing_mask_value[1], (erasing_height, erasing_width, C) ) X_copy[batch_index, erasing_left_top_y:erasing_left_top_y + erasing_height, erasing_left_top_x:erasing_left_top_x + erasing_width, :] = erasing_values return X_copy
random erasingの処理自体は、工夫することでforループをなくす形式でもっと高速にできたり、マスクがはみ出す場合の処理を効率的にする余地がありますが、今回はこの実装で動かしてみます。
描画用関数の準備
こちらは前回とほとんど同じ処理です。一部、MyImageDataGenerator
用に少し改変しています。
def plot_augmentation_image(train_image_sample, train_label_sample, params): # 同じデータを16個複製する train_image_samples = np.repeat( train_image_sample.reshape((1, *train_image_sample.shape)), 16, axis=0) train_label_sample = np.repeat( train_label_sample.reshape((1, *train_label_sample.shape)), 16, axis=0) # 16個に対してparamsで与えられた変換を実施 data_generator = MyImageDataGenerator(**params) generator = data_generator.flow( x=train_image_samples, y=train_label_sample, batch_size=16) # 変換後のデータを取得 batch_x, batch_y = generator.__next__() # 変換後はfloat32となっているため、uint8に変換 batch_x = batch_x.astype(np.uint8) # 描画処理 plt.figure(figsize=(10,10)) for i in range(16): plt.subplot(4,4,i+1) plt.imshow(batch_x[i]) plt.tick_params(labelbottom='off') plt.tick_params(labelleft='off')
描画して動作確認
まずは元論文でベースラインとして設定されているパラメータで検証します。
params = { 'random_erasing_probability': 0.5, 'random_erasing_area_ratio': [0.02, 0.4], 'random_erasing_aspect_ratio': [0.3, 1/0.3], 'random_erasing_mask_value': [0, 255], } plot_augmentation_image(train_image_sample, train_label_sample, params)
random_erasing_probability
の設定どおり、約半数の画像がマスクされています。
マスク値はランダムで埋めており、元論文でも平均値や固定値等いろいろ試行錯誤されているものの、ランダムをベースラインとして使用しているようです。
例えば、ramdon_erasing_probability
を100%にし、領域は元画像の半分まで、アスペクト比は正方形にしてみます。
params = { 'random_erasing_probability': 1.0, 'random_erasing_area_ratio': [0.5, 0.5], 'random_erasing_aspect_ratio': [1.0, 1.0], 'random_erasing_mask_value': [0, 255], } plot_augmentation_image(train_image_sample, train_label_sample, params)
設定どおりに変更されていることが確認できます。
トレーニング
前回同様、サンプルモデルで学習してみます。
まずはgeneratorを作成します。
params = { 'random_erasing_probability': 0.5, 'random_erasing_area_ratio': [0.02, 0.4], 'random_erasing_aspect_ratio': [0.3, 1/0.3], 'random_erasing_mask_value': [0, 255], } data_generator = MyImageDataGenerator(**params) batch_size = 32 generator = data_generator.flow(x=train_images, y=train_labels, batch_size=32)
モデル定義は前回と同様です。
model = keras.Sequential([ keras.layers.Conv2D(32, (3, 3), input_shape=(32, 32, 3), padding='same'), keras.layers.ReLU(), keras.layers.MaxPooling2D((2, 2)), keras.layers.Conv2D(32, (3, 3), padding='same'), keras.layers.ReLU(), keras.layers.MaxPooling2D((2, 2)), keras.layers.GlobalAveragePooling2D(), keras.layers.Dense(10, activation='softmax'), ])
学習実行時のみ、fit
関数にgeneratorを渡すことになるため注意が必要です。
model.compile( optimizer='Adam', loss='sparse_categorical_crossentropy', metrics=['accuracy'] ) model.fit(generator, epochs=3, steps_per_epoch=len(train_images) // batch_size)
カスタマイズしない場合は、fit
関数にiteratorを渡していましたが、今回のカスタマイズによりgeneratorを渡す形となっているため、step_per_epoch
で、エポックの終わりを明示的に指定する必要があります。
まとめ
いかがでしたでしょうか?画像のデータ拡張手法は、色々と新しい手法が開発されますので、カスタマイズを自身で実装する場合もあるかと思います。そんな時に本記事がお役に立てば幸いです。
データ拡張には複数画像を使うケースなどもありますので、機会があれば今後別のデータ拡張手法のカスタマイズについても紹介したいと思います。