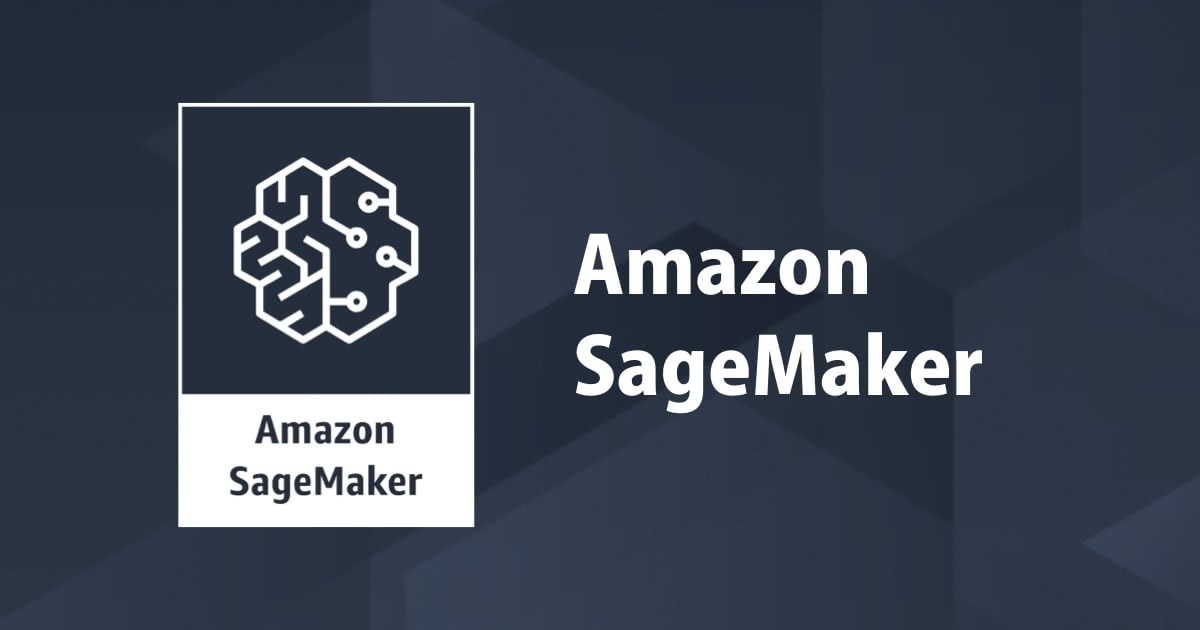
Amazon SageMaker Neoで画像分類モデルを最適化してみる – Amazon SageMaker Advent Calendar 2018
こんにちは、大阪DI部の大澤です。
この記事は「クラスメソッド Amazon SageMaker Advent Calendar」の8日目の記事となります。
今回はAmazon SageMakerで画像分類モデルを作成し、Amazon SageMaker Neoを使った画像分類モデルの最適化を試してみます。
Amazon SageMaker Neoとは
Amazon SageMaker Neoを使うことで機械学習モデルをコンパイルすることが出来ます。モデルのコンパイルを行うことで特定のデバイスに最適化を行うことが出来、推論速度の高速化と省メモリ化を実現できます。また、様々な機械学習フレームワークに対応しているためシステムのフレームワーク依存を減らすことが出来ます。Amazon SageMaker Neoの公式ページによると、今後Apache Software Licenseの下でOSS化も予定されているようです。
Amazon SageMaker Neoの公式ページより
Amazon SageMaker Neoを構成するコンポーネント
Amazon SageMaker Neoには2つの主要なコンポーネントがあります。
- コンパイラ
- モデルをフレームワークに依存しない中間表現に変換し、そこから最適化処理を施し、アーティファクトを出力します。
- アーティファクトにはモデルの定義、パラメータ、共有のオブジェクトライブラリが含まれます。
- ランタイム
- コンパイラが生成したアーティファクトを読み込んで、モデルを制御します。
対応状況
- 使用可能なリージョン:
- バージニア北部、オレゴン、オハイオ、アイルランド
- 対応するモデル:
- MXNet および TensorFlow でトレーニングされた AlexNet、ResNet、VGG、Inception、MobileNet、SqueezeNet、DenseNetなどのモデル
- XGBoostでトレーニングされたランダムカットフォレストモデルなど
- 対応するプラットフォーム:
- SageMaker ML.C5、ML.C4、ML.M5、ML.M4、ML.P3、ML.P2 インスタンス
- AWS DeepLens、Raspberry Pi、Jetson TX1 および TX2 デバイス
- Greengrass デバイスベースの Intel® Atom および Intel® Xeon CPU、 ARM Cortex-A CPU、Nvidia Maxwell および Pascal GPU
Amazon SageMakerのよくある質問が非常に参考になります。そのほかの情報や詳細についてはそちらをご覧ください。
やってみる
では、実際にやってみて理解を深めたいと思います。
セットアップ
SageMakerでモデルの学習やモデルをホストするエンドポイントを作成するために、その権限を持ったIAMロールが必要となるので、指定しておきます。 合わせて、学習に使用するデータや学習結果を保存する際に利用するS3の場所も定めておきます。
import sagemaker from sagemaker import get_execution_role role = get_execution_role() sess = sagemaker.Session() bucket='osawa-test-mv' prefix = 'ic'
データのダウンロードとアップロード
今回はCaltech256という画像分類用のデータセットを使います。こちらの学習用と検証用それぞれをダウンロードします。今回はSageMakerやMXNetで使える形にデータがすでに加工されたものをMXNetのファイル共有サイトからダウンロードしてきます。
import os import urllib.request import boto3 def download(url): filename = url.split("/")[-1] if not os.path.exists(filename): urllib.request.urlretrieve(url, filename) def upload_to_s3(channel, file): s3 = boto3.resource('s3') data = open(file, "rb") key = channel + '/' + file s3.Bucket(bucket).put_object(Key=key, Body=data) # 学習用と検証用それぞれダウンロード download('http://data.mxnet.io/data/caltech-256/caltech-256-60-train.rec') download('http://data.mxnet.io/data/caltech-256/caltech-256-60-val.rec')
S3にダウンロードしてきたデータをそのままアップロードします。
# S3での保存場所定義 s3train = 's3://{}/{}/train/'.format(bucket, prefix) s3validation = 's3://{}/{}/validation/'.format(bucket, prefix) # アップロード !aws s3 cp caltech-256-60-train.rec $s3train --quiet !aws s3 cp caltech-256-60-val.rec $s3validation --quiet
データの入力形式についてはドキュメントをご覧ください。
学習
画像分類用モデルを学習させます。
まずはモデルの学習をハンドルするEstimatorオブジェクトを作成します。 ここでは使用するIAMロールや学習用イメージ、学習用インスタンスデータなど学習に必要な情報を設定します。
s3_output_location = 's3://{}/{}/output'.format(bucket, prefix) ic = sagemaker.estimator.Estimator(training_image, role, train_instance_count=1, train_instance_type='ml.p3.8xlarge', train_volume_size = 50, train_max_run = 360000, input_mode= 'File', output_path=s3_output_location, sagemaker_session=sess)
次にハイパーパラメータの設定を行います。
サンプルノートブックではレイヤー数が18層になっていましたが、今回は152層に変更します。SageMaker Neoによるコンパイル効果を比較しやすくするためです。 今回は精度を求める訳ではないので、エポック数も1に変更します。
ic.set_hyperparameters(num_layers=152, # レイヤー数 image_shape = "3,224,224",# 画像サイズ num_classes=257, # クラス数 num_training_samples=15420, # 学習データ数 mini_batch_size=128, # ミニバッチ(一度に学習させる単位)の画像枚数 epochs=1, # エポック数(同じデータを何回学習させるか) learning_rate=0.01, # 学習率 top_k=2, # レポートされる精度の計算方法(上位何個のラベルのなかに答えのラベルがあれば正解とするか) precision_dtype='float32' # 計算時の精度。精度を落とすことで計算量が減る )
データチャネルごとにデータがどこにあるかを指定します。
train_data = sagemaker.session.s3_input(s3train, distribution='FullyReplicated', content_type='application/x-recordio', s3_data_type='S3Prefix') validation_data = sagemaker.session.s3_input(s3validation, distribution='FullyReplicated', content_type='application/x-recordio', s3_data_type='S3Prefix') data_channels = {'train': train_data, 'validation': validation_data}
学習を開始します!
ic.fit(inputs=data_channels, logs=True)
通常のモデル
まずはSageMaker Neoでコンパイルせずにモデルをデプロイし、推論を試してみます。
モデルのデプロイ
モデルをホストするエンドポイントを作成します。 サンプルノートブックではc5.4xlargeになっていましたが、c5.xlargeに変更します。レイヤー数と同様、推論時間をより長くするためです。
ic_classifier = ic.deploy(initial_instance_count = 1, instance_type = 'ml.c5.xlarge')
推論
テスト画像をCaltech256からダウンロードしてきます。
!wget -O /tmp/test.jpg http://www.vision.caltech.edu/Image_Datasets/Caltech256/images/080.frog/080_0001.jpg file_name = '/tmp/test.jpg' # テスト画像を見てみる from IPython.display import Image Image(file_name)
カエルの写真です。
推論リクエストとして投げるために画像をバイナリに変換します。あわせて、推論時の入力データ形式も設定します。
import json import numpy as np with open(file_name, 'rb') as f: payload = f.read() payload = bytearray(payload) ic_classifier.content_type = 'application/x-image'
ではエンドポイントに推論リクエストを投げます。 サンプルノートブックから処理を変えて、リクエストを100回投げてその平均時間等を計算してみます。
%%time import time import pandas as pd process_times = [] for i in range(100): start_time = time.time() result = json.loads(ic_classifier.predict(payload)) process_times.append(time.time() - start_time) print(pd.Series(process_times).describe())
推論の結果は各クラスごとに確率が入っているので、その確率が最大のものを取り出すことで推論結果を確認できます。今回はモデルの学習をしっかりとやった訳ではないので、精度は良くないです。 では、先ほど100回投げていた推論リクエストの最後の一回の結果を見てみます。
# the result will output the probabilities for all classes # find the class with maximum probability and print the class index index = np.argmax(result) object_categories = ['ak47', 'american-flag', 'backpack', 'baseball-bat', 'baseball-glove', 'basketball-hoop', 'bat', 'bathtub', 'bear', 'beer-mug', 'billiards', 'binoculars', 'birdbath', 'blimp', 'bonsai-101', 'boom-box', 'bowling-ball', 'bowling-pin', 'boxing-glove', 'brain-101', 'breadmaker', 'buddha-101', 'bulldozer', 'butterfly', 'cactus', 'cake', 'calculator', 'camel', 'cannon', 'canoe', 'car-tire', 'cartman', 'cd', 'centipede', 'cereal-box', 'chandelier-101', 'chess-board', 'chimp', 'chopsticks', 'cockroach', 'coffee-mug', 'coffin', 'coin', 'comet', 'computer-keyboard', 'computer-monitor', 'computer-mouse', 'conch', 'cormorant', 'covered-wagon', 'cowboy-hat', 'crab-101', 'desk-globe', 'diamond-ring', 'dice', 'dog', 'dolphin-101', 'doorknob', 'drinking-straw', 'duck', 'dumb-bell', 'eiffel-tower', 'electric-guitar-101', 'elephant-101', 'elk', 'ewer-101', 'eyeglasses', 'fern', 'fighter-jet', 'fire-extinguisher', 'fire-hydrant', 'fire-truck', 'fireworks', 'flashlight', 'floppy-disk', 'football-helmet', 'french-horn', 'fried-egg', 'frisbee', 'frog', 'frying-pan', 'galaxy', 'gas-pump', 'giraffe', 'goat', 'golden-gate-bridge', 'goldfish', 'golf-ball', 'goose', 'gorilla', 'grand-piano-101', 'grapes', 'grasshopper', 'guitar-pick', 'hamburger', 'hammock', 'harmonica', 'harp', 'harpsichord', 'hawksbill-101', 'head-phones', 'helicopter-101', 'hibiscus', 'homer-simpson', 'horse', 'horseshoe-crab', 'hot-air-balloon', 'hot-dog', 'hot-tub', 'hourglass', 'house-fly', 'human-skeleton', 'hummingbird', 'ibis-101', 'ice-cream-cone', 'iguana', 'ipod', 'iris', 'jesus-christ', 'joy-stick', 'kangaroo-101', 'kayak', 'ketch-101', 'killer-whale', 'knife', 'ladder', 'laptop-101', 'lathe', 'leopards-101', 'license-plate', 'lightbulb', 'light-house', 'lightning', 'llama-101', 'mailbox', 'mandolin', 'mars', 'mattress', 'megaphone', 'menorah-101', 'microscope', 'microwave', 'minaret', 'minotaur', 'motorbikes-101', 'mountain-bike', 'mushroom', 'mussels', 'necktie', 'octopus', 'ostrich', 'owl', 'palm-pilot', 'palm-tree', 'paperclip', 'paper-shredder', 'pci-card', 'penguin', 'people', 'pez-dispenser', 'photocopier', 'picnic-table', 'playing-card', 'porcupine', 'pram', 'praying-mantis', 'pyramid', 'raccoon', 'radio-telescope', 'rainbow', 'refrigerator', 'revolver-101', 'rifle', 'rotary-phone', 'roulette-wheel', 'saddle', 'saturn', 'school-bus', 'scorpion-101', 'screwdriver', 'segway', 'self-propelled-lawn-mower', 'sextant', 'sheet-music', 'skateboard', 'skunk', 'skyscraper', 'smokestack', 'snail', 'snake', 'sneaker', 'snowmobile', 'soccer-ball', 'socks', 'soda-can', 'spaghetti', 'speed-boat', 'spider', 'spoon', 'stained-glass', 'starfish-101', 'steering-wheel', 'stirrups', 'sunflower-101', 'superman', 'sushi', 'swan', 'swiss-army-knife', 'sword', 'syringe', 'tambourine', 'teapot', 'teddy-bear', 'teepee', 'telephone-box', 'tennis-ball', 'tennis-court', 'tennis-racket', 'theodolite', 'toaster', 'tomato', 'tombstone', 'top-hat', 'touring-bike', 'tower-pisa', 'traffic-light', 'treadmill', 'triceratops', 'tricycle', 'trilobite-101', 'tripod', 't-shirt', 'tuning-fork', 'tweezer', 'umbrella-101', 'unicorn', 'vcr', 'video-projector', 'washing-machine', 'watch-101', 'waterfall', 'watermelon', 'welding-mask', 'wheelbarrow', 'windmill', 'wine-bottle', 'xylophone', 'yarmulke', 'yo-yo', 'zebra', 'airplanes-101', 'car-side-101', 'faces-easy-101', 'greyhound', 'tennis-shoes', 'toad', 'clutter'] print("Result: label - " + object_categories[index] + ", probability - " + str(result[index]))
正解はカエルのはずがクマと推論されました。
エンドポイントの削除
通常のモデルをホストしているエンドポイントは不要になったので、削除します。
ic_classifier.delete_endpoint()
最適化モデル
次に、SageMaker Neoでコンパイルして最適化したモデルで推論を試してみます。
モデルのコンパイル
SageMaker Neoでモデルをコンパイルします。その際に以下のようなパラメータが必要になります。
- target_instance_family: モデルを最適化したいインスタンスファミリー
- input_shape: 入力データ形式
- output_path: コンパイルしたモデルを出力する場所(S3)
- framework: フレームワーク(mxnetやtensorflowなど)
- framework_version: フレームワークのバージョン
詳細についてはドキュメントをご覧ください。
output_path = '/'.join(ic.output_path.split('/')[:-1]) #モデルをコンパイルする。戻り値はsagemaker.model.Model optimized_ic = ic.compile_model(target_instance_family='ml_c5', input_shape={'data':[1, 3, 224, 224]}, # Batch size 1, 3 channels, 224x224 Images. output_path=output_path, framework='mxnet', framework_version='1.2.1')
画像分類用のコンテナイメージのURIを誤ったものを設定するという既知のバグがあるようなので、設定し直します。モデル名も同様に設定し直します。
# There is a known issue where SageMaker SDK locates the incorrect docker image URI for Image Classification # For now, we manually set Image URI optimized_ic.image = get_image_uri(sess.boto_region_name, 'image-classification-neo', repo_version="latest") # There is a known issue where SageMaker SDK does not set the same. In the mean time we set the name optimized_ic.name = 'deployed-image-classification'
エンドポイントの作成
最適化したモデルをホストするエンドポイントを作成します。
optimized_ic_classifier = optimized_ic.deploy(initial_instance_count = 1, instance_type = 'ml.c5.4xlarge') optimized_ic_classifier.content_type = 'application/x-image'
推論
エンドポイントに推論リクエストを投げます。 通常のモデルと同様に100回のリクエストを投げて、時間を計測します。
%%time import time import pandas as pd process_times = [] for i in range(100): start_time = time.time() result = json.loads(optimized_ic_classifier.predict(payload)) process_times.append(time.time() - start_time) print(pd.Series(process_times).describe())
今回試した結果を通常のモデルと比べて推論にかかった時間を平均値で比較すると、およそ30%ほど高速化しています。
※モデルの最適化による高速化の効果はモデルの種類やデータによって大いに変わると思います。今回試しているサンプルノートブックに従ってのままだと、モデルの最適化前後共に50~100msくらいでした。コンパイル(最適化)によってオーバーヘッドがあるためか、最適化後の方がリクエスト速度が遅かったです。
最適化前(モデルのレイヤー数:18、推論エンドポイントのインスタンスタイプ:c5.4xlarge)
最適化後(モデルのレイヤー数:18、推論エンドポイントのインスタンスタイプ:c5.4xlarge)
エンドポイントの削除
エンドポイントが不要になったら、無駄なコストがかからないように
optimized_ic_classifier.delete_endpoint()
さいごに
今回は 「クラスメソッド Amazon SageMaker Advent Calendar」 の8日目として、「Amazon SageMaker Neoを使った画像分類モデルの最適化」についてお送りしました。Amazon SageMaker Neoを使ってモデルを環境に合わせて最適化することによって、推論速度の高速化を見込めます。APIにしてもバッチ処理にしても一件あたりの推論速度を改善することで、エンドポイント一台あたりの処理可能数を増やしたり、全体の処理時間を短くすることが出来ます。東京リージョンの対応とOSS化が楽しみです!
お読みくださりありがとうございました〜!明日もお楽しみに〜!