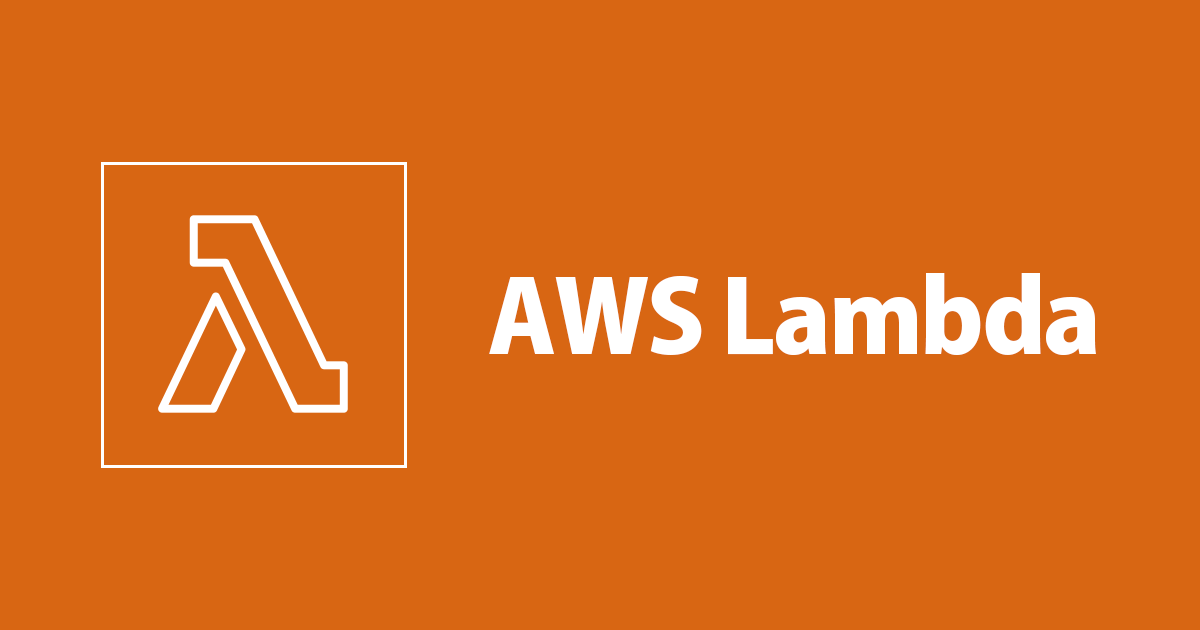
AWS LambdaとPyArrow3.0.0を使ってサクッとParquetファイルに変換する
Apache Arrowは目覚ましい進化を遂げて、今年の1月にVersion3.0がリリースされ、今月から最新のpyarrow 3.0.0もpipコマンドで普通にインストールできるようになりました。以前ブログで取り上げた2年前のpyarrow 0.13.0は、decimal型やdate型のサポートされていませんでした。
本日は、2年前と同じテーマですが、最新のApache Arrow(PyArrow3.0.0)とLambda Functionを使って、S3にファイル出力したイベントで即座にParquetに変換してデータレイクへ配置する方法をご紹介します。
Apache Arrow(PyArrow)とは
Apache Arrowは、インメモリの列指向データフォーマットです。Hadoop/Spark(Hadoopのエコシステム)やPandas(PyDataのエコシステム)をはじめとするツール間でゼロコピーデータアクセスを目指し、Apache Arrowがその共通データフォーマットになるように設計されています。つまり、Apache Arrowに読み込むと他のツールとデータが共有できたり、様々なデータファイルフォーマットに出力できるようになります。
PyArrow3.0.0によるParquetファイルに変換
PyArrowから直接CSVファイルを読み込んでParquet出力する方法を用いて変換し、サポートしているデータ型をそれぞれ検証します。
サポートしているデータ型
Amazon AthenaとAmazon Redshift Spectrumの両方でサポートしているデータ型の主要なデータ型が、Apache Arrow(PyArrow3.0.0)でサポートされています。
※ Apache Arrowがサポートしている全てのデータ型は、Data Types and Schemasをご覧ください。
Python3 | PyArrow | Amazon Athena | Amazon Redshift Spectrum | 対応状況 |
---|---|---|---|---|
int | int16 | smallint | smallint | ○ |
int | int32 | int | integer | ○ |
int | int64 | bigint | bigint | ○ |
float | float32 | float | real | ○ |
float | float64 | double | double precision | ○ |
decimal | decimal | decimal(x, y) | decimal | ○ |
bool | bool_ | boolean | boolean | ○ |
str | string | string | varchar(65535) | ○ |
str | string | char(n) | char(n) | ○ |
str | string | varchar(n) | varchar(n) | ○ |
date | date | date | date | ○ |
datetime | timestamp | timestamp | timestamp | ○ |
サンプルデータ
今回は、この組み合わせを試すために以下のカラムのデータ(sales.tsv)を用意しました。
No | カラム名 | PyArroのデータ型の定義 | 実際のデータ |
---|---|---|---|
1 | id | int16() | 1 |
2 | price | int32() | 20000 |
3 | total | int64() | 300000000 |
4 | price_profit | float32() | 4.56 |
5 | total_profit | float64() | 67.89 |
6 | discount | decimal(10, 2) | 789012.34 |
7 | visible | bool_() | True |
8 | name | string() | QuietComfort 35 |
9 | created | date32() | 2019-06-14 |
10 | updated | timestamp('s') | 2019-06-14 23:59:59 |
id price total price_profit total_profit discount visible name created updated 1 20000 300000000 4.56 67.89 789012.34 True QuietComfort 35 2019-06-14 2019-06-14 23:59:59
PyArrow3.0.0用のLambda Layerを作成する
Lambda動作環境の選定
今回は、TSVファイルに軽量・高速に変換するためAWS Lambdaを用います。Lambdaは、パッケージフォーマットとして、従来どおりLambda関数を用いる方法に加えて、コンテナイメージがサポートされました。複数のLambdaアプリケーションや関数から再利用されることを考慮して、デプロイパッケージは、Layerを用います。
Lambdaの制約事項
デプロイパッケージを問わず、以下の制約がありますので、このリソース条件で変換するように実装します。
- ローカルのストレージ(/tmp)は512MB
- メモリは最大10GB(メモリ10GBの場合は、vCPU x 6)
現在のPyArrow3.0.0は、S3から直接読み込みはサポートされていませんので、boto3で/tmpにコピーしたファイルをarrow形式でメモリに読み込んだ後、Parquet形式で/tmpに書き出してS3のデータレイクストレージにboto3でコピーします。そのため、入出力ファイルは512MBが上限になります。この上限を超えたいのなら、EFSでストレージをマウントするなどご検討ください。
Lambda Layerを作成
作成するために最新のAmazon Linux2のEC2インスタンス上で作成します。今日現在のEC2インスタンスのPython3のデフォルトバージョンであるPython3.7(3.7.9)をインストールします。
- Amazon Linux 2(t3.nano)
- Python 3 (3.7.9)
コンパイラや開発用ライブラリを事前にインストールして準備します。
[ec2-user@ip-10-0-0-247 ~]$ sudo yum install gcc gcc-c++ kernel-devel python-devel libxslt-devel libffi-devel openssl-devel 読み込んだプラグイン:extras_suggestions, langpacks, priorities, update-motd amzn2-core | 3.7 kB 00:00:00 パッケージ python-devel-2.7.18-1.amzn2.0.3.x86_64 はインストール済みか最新バージョンです 依存性の解決をしています --> トランザクションの確認を実行しています。 ---> パッケージ gcc.x86_64 0:7.3.1-12.amzn2 を インストール : : 完了しました!
本題のLambda Layerを作成します。Layerのランタイムのサイズは130MBです。(Layerのランタイムの展開後の最大サイズは150MB未満)
[ec2-user@ip-10-0-0-247 ~]$ mkdir python [ec2-user@ip-10-0-0-247 ~]$ pip3 install -t ./python pyarrow [ec2-user@ip-10-0-0-247 ~]$ ll python/ 合計 8 drwxrwxr-x 2 ec2-user ec2-user 66 3月 23 07:41 bin drwxrwxr-x 18 ec2-user ec2-user 4096 3月 23 07:41 numpy drwxrwxr-x 2 ec2-user ec2-user 158 3月 23 07:41 numpy-1.20.1.dist-info drwxrwxr-x 2 ec2-user ec2-user 154 3月 23 07:41 numpy.libs drwxrwxr-x 7 ec2-user ec2-user 4096 3月 23 07:41 pyarrow drwxrwxr-x 2 ec2-user ec2-user 165 3月 23 07:41 pyarrow-3.0.0.dist-info [ec2-user@ip-10-0-0-247 ~]$ du -s python 130412 python [ec2-user@ip-10-0-0-247 ~]$ zip -r pyarrow.zip python adding: python/ (stored 0%) adding: python/numpy.libs/ (stored 0%) adding: python/numpy.libs/libz-eb09ad1d.so.1.2.3 (deflated 50%) : : adding: python/bin/f2py3.7 (deflated 25%) adding: python/bin/plasma_store (deflated 31%) [ec2-user@ip-10-0-0-247 ~]$ ll pyarrow.zip -rw-rw-r-- 1 ec2-user ec2-user 39123022 3月 23 07:42 pyarrow.zip
このランタイム(pyarrow.zip)をpyarrow
というレイヤ名で登録します。
Lambda関数の作成
データの型指定
今回用いるデータ型を先頭でインポートしています。column_typesにカラム名とデータ型をKEY/VALUEで指定します。上記の一覧表に記載したデータ型をすべて指定しています。
from pyarrow import int16, int32, int64, float64, float32, bool_, date32, date64, decimal128, timestamp, string, Table, parquet as pq : : column_types = { 'id': int16(), 'price': int32(), 'total': int64(), 'price_profit': decimal128(5,2), 'total_profit': float32(), 'discount': float64(), 'visible': bool_(), 'name': string(), 'created': date32(), 'updated': timestamp('s') }
入力データの読み込み
- ReadOptions()
- スレッド数、ブロックサイズ、メモリプール、などの入力ファイルの読み込みの挙動を指定します。
- (?)このTSVファイルは、先頭はヘッダ行なので、
skip_rows=1
が必要だが指定しなくてもスキップされた。
- ParseOptions()
- 入力ファイルのフォーマットを指定します。
- ConvertOptions()
- 入力データのデータ型指定、スキーマ検証、NULLの取り扱いなどを指定します。
- read_csv()
- 入力ファイルパスや上記のオプションを指定して、arrow形式のメモリに読みます。
- tsvの場合は
read_csv()
ですが、jsonの場合はread_json()
を用います。
def get_pyarrow_table(input_files: str, column_types: dict) -> Table: readoptions = ReadOptions( use_threads=True, # 複数の読み取りスレッドの利用 block_size=1000 # 読み取りブロック数 ) parseoptions = ParseOptions( delimiter='\t', # タブ区切り指定 double_quote=False, # ダブルクオートで括らない escape_char='\'', # エスケープ文字の指定 ) convertoptions = ConvertOptions( check_utf8=True, # 文字列カラムのUTF-8妥当性をチェック column_types=column_types, # 列のデータ型を辞書型で渡す null_values=[''] # データ内のNULLを表す文字列 ) pyarrow_table = read_csv( input_file=input_files, read_options=readoptions, parse_options=parseoptions, convert_options=convertoptions ) return pyarrow_table
出力データの書き込み
arrow形式のメモリを指定したファイル形式やオプションに従い、Parquetファイル出力します。
pq.write_table( arrow_table, output_file, compression='snappy', # snappyで圧縮 flavor=['spark'], # spark互換の設定 version='1.0', )
デプロイした関数
実際にデプロイしたLambda関数(S3EventCSVtoParquet
)は以下のとおりです。
import os import urllib.parse from pyarrow.csv import read_csv, ReadOptions, ParseOptions, ConvertOptions from pyarrow import int16, int32, int64, float64, float32, bool_, date32, date64, decimal128, timestamp, string, Table, parquet as pq from botocore.exceptions import ClientError import boto3 # # Functions # def get_pyarrow_table(input_files: str, column_types: dict) -> Table: readoptions = ReadOptions( use_threads=True, # 複数の読み取りスレッドの利用 block_size=1000 # 読み取りブロック数 ) parseoptions = ParseOptions( delimiter='\t', # タブ区切り指定 double_quote=False, # ダブルクオートで括らない escape_char='\'', # エスケープ文字の指定 ) convertoptions = ConvertOptions( check_utf8=True, # 文字列カラムのUTF-8妥当性をチェック column_types=column_types, # 列のデータ型を辞書型で渡す null_values=[''] # データ内のNULLを表す文字列 ) pyarrow_table = read_csv( input_file=input_files, read_options=readoptions, parse_options=parseoptions, convert_options=convertoptions ) return pyarrow_table def tsv2parquet(input_file: str, output_file: str, column_types: dict): arrow_table = get_pyarrow_table( input_file, column_types ) if os.path.exists(input_file): os.remove(input_file) pq.write_table( arrow_table, output_file, compression='snappy', # snappyで圧縮 flavor=['spark'], # spark互換の設定 version='1.0', ) def get_target_key(source_key: str): source_tok = source_key.split('/') source_file = source_tok[-1] file_prefix = source_file.split('.')[0] target_key = source_tok[0] + '/target/' + file_prefix + '.parquet' return target_key def lambda_handler(event, context): source_bucket = event['Records'][0]['s3']['bucket']['name'] source_key = urllib.parse.unquote_plus(event['Records'][0]['s3']['object']['key'], encoding='utf-8') s3resource = boto3.resource('s3') target_bucket = source_bucket target_key = get_target_key(source_key) source_files = '/tmp/' + source_key.split('/')[-1] target_file = '/tmp/' + target_key.split('/')[-1] try: s3resource.Bucket(source_bucket).download_file(Filename=source_files, Key=source_key) column_types = { 'id': int16(), 'price': int32(), 'total': int64(), 'price_profit': decimal128(5,2), 'total_profit': float32(), 'discount': float64(), 'visible': bool_(), 'name': string(), 'created': date32(), 'updated': timestamp('s') } tsv2parquet(source_files, target_file, column_types) s3resource.Bucket(target_bucket).upload_file(Filename=target_file, Key=target_key) if os.path.exists(target_file): os.remove(target_file) except ClientError as e: print(e)
S3イベントの設定
上記のLambda LayerとLambda関数と登録した後、入力データファイルのS3バケットとPrefix、Suffixを指定して、オブジェクト作成イベント(All object create events
)で、このLambda関数を起動するイベントを登録します。あとは、入力ファイルをS3にコピーすると自動的にLambda関数が呼び出され、Parquetファイルが出力されます。
動作検証
変換結果の確認
Parquetにフォーマット変換されたsales.parquet
をGlue Crawlerで自動認識させてクエリした結果は以下のとおり、正しく変換されました。
もちろん、AthenaのDDLからもその結果が確認できます。
CREATE EXTERNAL TABLE `target`( `id` smallint, `price` int, `total` bigint, `price_profit` float, `total_profit` double, `discount` decimal(10,2), `visible` boolean, `name` string, `created` date, `updated` timestamp) ROW FORMAT SERDE 'org.apache.hadoop.hive.ql.io.parquet.serde.ParquetHiveSerDe' STORED AS INPUTFORMAT 'org.apache.hadoop.hive.ql.io.parquet.MapredParquetInputFormat' OUTPUTFORMAT 'org.apache.hadoop.hive.ql.io.parquet.MapredParquetOutputFormat' LOCATION 's3://cm-bucket/pyarrowutil/target/' TBLPROPERTIES ( 'CrawlerSchemaDeserializerVersion'='1.0', 'CrawlerSchemaSerializerVersion'='1.0', 'UPDATED_BY_CRAWLER'='sales', 'averageRecordSize'='778', 'classification'='parquet', 'compressionType'='none', 'objectCount'='1', 'recordCount'='1', 'sizeKey'='3528', 'typeOfData'='file')
パフォーマンス検証
実用性を検証するため、上記のソースを書き換えて約100MBデータ(customer0002_part_00.gz)をParquetファイルに変換してみます。
メモリとタイムアウトの変更
データサイズが大きいので、メモリを128MBから5120MB、タイムアウトを3秒から3分に変更しました。(この値は、あくまでも私の動物の「感」で、何の根拠もありません。)
カラムの定義の変更
column_types = { 'c_custkey': int32(), 'c_name': string(), 'c_address': string(), 'c_city': string(), 'c_nation': string(), 'c_region': string(), 'c_phone': string(), 'c_mktsegment': string(), }
入力フォーマットの定義
PSVファイルは、先頭からデータ行なので、skip_rows=0
などつけなくても済むはずだが、先頭行はカラム名として設定されてしまう現象が発生。そのため、columnnames_optionsをReadOptionsにcolumnnames_options指定して、カラム名を設定しています。
また、今回はPSV(パイプ文字区切りファイル)のため、delimiter='|'
と指定しています。
def get_pyarrow_table(input_files: str, column_types: dict) -> Table: columnnames_options = [ 'c_custkey', 'c_name', 'c_address', 'c_city', 'c_nation', 'c_region', 'c_phone', 'c_mktsegment', ] readoptions = ReadOptions( use_threads=True, # 複数の読み取りスレッドの利用 block_size=1000, # 読み取りブロック数 column_names=columnnames_options, #column_names ) parseoptions = ParseOptions( delimiter='|', # パイプ文字区切り指定 double_quote=False, # ダブルクオートで括らない escape_char='\'', # エスケープ文字の指定 ) : : return pyarrow_table
変換結果(100MBの.gzファイルの変換)
ファイルサイズ
変換のみなら、この圧縮ファイル(customer0002_part_00.gz)100MBを展開すると286.5MBあり、変換後のParquetファイルは118.4MBでした。
処理時間
- 30.01秒:入出力ファイルの変換のみ
- 33.47秒:入出力ファイルの変換、S3のダウンロード・アップロード
AWS利用費の試算
5,120MB(0.0000000833USD/ms)なので、AWS料金を試算すると0.002788051(USD)です。Glueと比較すると圧倒的な速さと安さです。
0.002788051(USD) = 0.0000000833 ✕ 1000 ✕ 33.47
まとめ
2年前のpyarrow 0.13.0は、decimal型やdate型のサポートされていませんでしたが、pyarrow 3.0.0では、主要なデータ型をサポートし、AthenaやRedshift Spectrumが正常に認識できるParquetファイルが生成できることを確認できました。
これまではAWS GlueのETLジョブを用いてParquetを生成していましたが、単純なフォーマット変換だけであれば、AWS LambdaとPyArrowの組み合わせで代用できるようになり、データレイクなどのサーバレスアナリティクスが加速します。Glue(Spark)は、データを変換して、DataFrameをソートしたり、ParquetファイルをPartitioningやBucketingして出力も可能ですので、利用目的に応じて使い分けると良いでしょう。
データ分析界のスタープレーヤーが集まって生み出されたApache Arrowですが、まだまだ進化が終わる気配がありません。機能やファイルフォーマットサポートなど、今後も引き続きウオッチしたいと思います。