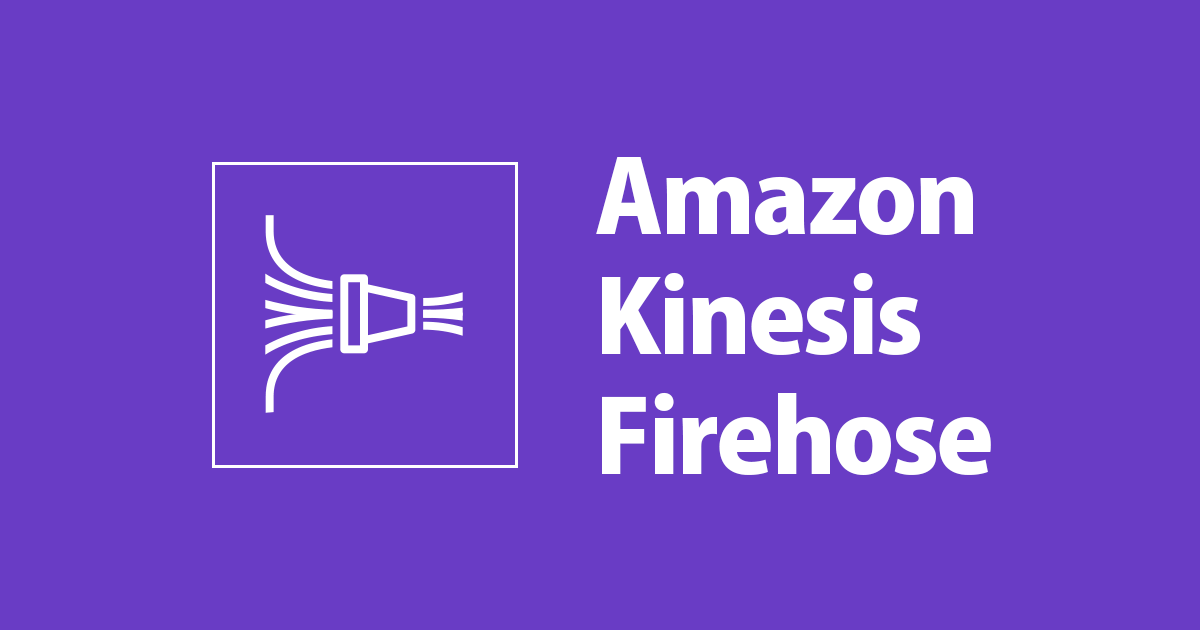
Firehoseで Parquet形式に変換したALBのアクセスログをAthenaで解析してみた
AWSチームのすずきです。
ALBのアクセスログ を Athena で効率の良い解析を行うため、 Lambda と Parquet形式への変換を有効にしたFirehose を利用する機会がありましたので、紹介させていただきます。
概要図
設定
今回のAWSリソース、CloudFormation で設置しました。主要な設定内容を紹介します。
利用したテンプレートは記事末尾にリンクします。
Glue
Firehoseの変換データの出力先となる Glue Table の設定を行います。
TableInput
入出力フォーマットは「Parquet」を利用する指定とします。
TableInput: Owner: owner InputFormat: org.apache.hadoop.hive.ql.io.parquet.MapredParquetInputFormat OutputFormat: org.apache.hadoop.hive.ql.io.parquet.MapredParquetOutputFormat SerdeInfo: SerializationLibrary: org.apache.hadoop.hive.ql.io.parquet.serde.ParquetHiveSerDe Parameters: classification: parquet
カラム
Athenaで数値集計を実施する項目は数値型、 所要時間(request_processing_time、target_processing_time、response_processing_time) は「double」、 転送容量(received_bytes、sent_bytes)は「bigint」と設定しました。
Columns: - Name: type Type: string - Name: time Type: string - Name: request_processing_time Type: double - Name: target_processing_time Type: double - Name: response_processing_time Type: double - Name: elb_status_code Type: string - Name: target_status_code Type: string - Name: received_bytes Type: bigint - Name: sent_bytes Type: bigint
Location、PartitionKeys
FirehoseのS3出力設定に合わせて設定します。
StorageDescriptor: Location: !Sub 's3://${S3bucketFirehose}/${GlueDbName}/${GlueTableName}/' PartitionKeys: - Name: dt Type: date - Name: hour Type: int
Firehose
Prefix
S3出力先のプリフィックスはカスタマイズ。日付(dt: %Y-%m-%d)と、時刻(hour: %H)を Athena/Glue の パーティションとする設定としました。
ExtendedS3DestinationConfiguration: BucketARN: !Sub 'arn:aws:s3:::${S3bucketFirehose}' Prefix: !Sub '${GlueDbName}/${GlueTableName}/dt=!{timestamp:YYYY}-!{timestamp:MM}-!{timestamp:dd}/hour=!{timestamp:HH}/' ErrorOutputPrefix: !Sub '${GlueDbName}/${GlueTableName}-error/!{firehose:error-output-type}/dt=!{timestamp:YYYY}-!{timestamp:MM}-!{timestamp:dd}/hour=!{ti
DataFormatConversionConfiguration
出力先となるGlueテーブルを指定、Firehoseへの入力形式はJSON「OpenXJsonSerDe」を指定しました。
DataFormatConversionConfiguration: SchemaConfiguration: CatalogId: !Ref 'AWS::AccountId' RoleARN: !GetAtt 'DeliveryRole.Arn' DatabaseName: !Ref 'GlueDatabase' TableName: !Ref 'GlueTable' Region: !Ref 'AWS::Region' VersionId: LATEST InputFormatConfiguration: Deserializer: OpenXJsonSerDe: {} OutputFormatConfiguration: Serializer: ParquetSerDe: {} Enabled: true
Lambda
Python 3.7 の Lambda関数を利用しました。ログのパースは以下記事を踏襲しています。
ETL
データの前加工を実施、Glueテーブルの定義に合わせます。
日付情報
日付型としてミリ秒をサポートしない QuickSight の利用を想定し、秒から小数点以下を除去した日付カラムを用意しました。
a["timestamp"] = datetime.strptime(a["time"], "%Y-%m-%dT%H:%M:%S.%fZ").strftime('%Y-%m-%dT%H:%M:%S')
数値
Glueテーブルの定義に合わせ「double」はPythonの「float」型、「bigint」は「int」型へ変換を行います。
数値型への変換に失敗するデータ(「-」など)は、出力対象から除外しFirehoseの変換エラーを回避します。
#float try: a["request_processing_time"] = float(a["request_processing_time"]) except: a.pop("request_processing_time") #int try: a["received_bytes"] = int(a["received_bytes"]) except: a.pop("received_bytes")
Firehose登録
「put_record_batch」、APIの上限 1000件、1MB を超過しないよう、300件、400KB毎にバッチ登録を実施します。
APIの戻り値でFirehoseへの登録失敗を示す「FailedPutCount」が含まれた場合、ログ出力のみ実施します。
def put_log_firehose(data): b = [] for a in data: b.append({'Data': json.dumps(a) + "\n"}) if len(b) > 300 or len(str(b)) > 400000: r = put_record_firehose(b) b = [] if len(b) > 0: r = put_record_firehose(b) def put_record_firehose(d): s = os.environ['firehose_stream_name'] r = firehose.put_record_batch( DeliveryStreamName = s, Records = d ) if r['FailedPutCount'] > 0: print (json.dumps(r))
- 東京リージョンのFirehoseに対して、毎秒1MB、1000件以上のログを投入する場合、事前に上限緩和申請が必要です。(2019年9月現在)
Amazon Kinesis Data Firehose の制限:
各 Kinesis Data Firehose 配信ストリームには以下の制限があります。 米国東部(バージニア北部)、米国西部 (オレゴン)、欧州 (アイルランド) の場合: 5,000 レコード/秒、2,000 トランザクション/秒、5 MiB/秒。
EU (パリ)、アジアパシフィック (ムンバイ)、米国東部 (オハイオ)、欧州 (フランクフルト)、南米 (サンパウロ)、アジアパシフィック (香港)、アジアパシフィック (ソウル)、欧州 (ロンドン)、アジアパシフィック (東京)、米国西部 (北カリフォルニア)、アジアパシフィック (シンガポール)、アジアパシフィック (シドニー)、AWS GovCloud (US-West)、AWS GovCloud (米国東部)、欧州 (ストックホルム)、カナダ (中部) の場合: 1,000 レコード/秒、1,000 トランザクション/秒、1 MiB/秒。
テストイベント
今回ALBのサンプルログをJSONに変換、Lambdaのテストイベントとして登録します。
[ "http 2018-07-02T22:23:00.186641Z app/my-loadbalancer/50dc6c495c0c9188 192.168.131.39:2817 10.0.0.1:80 0.000 0.001 0.000 200 200 34 366 \"GET http://www.example.com:80/ HTTP/1.1\" \"curl/7.46.0\" - - arn:aws:elasticloadbalancing:us-east-2:123456789012:targetgroup/my-targets/73e2d6bc24d8a067 \"Root=1-58337262-36d228ad5d99923122bbe354\" \"-\" \"-\" 0 2018-07-02T22:22:48.364000Z \"forward\" \"-\" \"-\"", "h2 2018-07-02T22:23:00.186641Z app/my-loadbalancer/50dc6c495c0c9188 10.0.1.252:48160 10.0.0.66:9000 0.000 0.002 0.000 200 200 5 257 \"GET https://10.0.2.105:773/ HTTP/2.0\" \"curl/7.46.0\" ECDHE-RSA-AES128-GCM-SHA256 TLSv1.2 arn:aws:elasticloadbalancing:us-east-2:123456789012:targetgroup/my-targets/73e2d6bc24d8a067 \"Root=1-58337327-72bd00b0343d75b906739c42\" \"-\" \"-\" 1 2018-07-02T22:22:48.364000Z \"redirect\" \"https://example.com:80/\" \"-\"", "https 2018-07-02T22:23:00.186641Z app/my-loadbalancer/50dc6c495c0c9188 192.168.131.39:2817 10.0.0.1:80 0.086 0.048 0.037 200 200 0 57 \"GET https://www.example.com:443/ HTTP/1.1\" \"curl/7.46.0\" ECDHE-RSA-AES128-GCM-SHA256 TLSv1.2 arn:aws:elasticloadbalancing:us-east-2:123456789012:targetgroup/my-targets/73e2d6bc24d8a067 \"Root=1-58337281-1d84f3d73c47ec4e58577259\" \"www.example.com\" \"arn:aws:acm:us-east-2:123456789012:certificate/12345678-1234-1234-1234-123456789012\" 1 2018-07-02T22:22:48.364000Z \"authenticate,forward\" \"-\" \"-\"", "wss 2018-07-02T22:23:00.186641Z app/my-loadbalancer/50dc6c495c0c9188 10.0.0.140:44244 10.0.0.171:8010 0.000 0.001 0.000 101 101 218 786 \"GET https://10.0.0.30:443/ HTTP/1.1\" \"-\" ECDHE-RSA-AES128-GCM-SHA256 TLSv1.2 arn:aws:elasticloadbalancing:us-west-2:123456789012:targetgroup/my-targets/73e2d6bc24d8a067 \"Root=1-58337364-23a8c76965a2ef7629b185e3\" \"-\" \"-\" 1 2018-07-02T22:22:48.364000Z \"forward\" \"-\" \"-\"", "http 2018-11-30T22:23:00.186641Z app/my-loadbalancer/50dc6c495c0c9188 192.168.131.39:2817 - 0.000 0.001 0.000 200 200 34 366 \"GET http://www.example.com:80/ HTTP/1.1\" \"curl/7.46.0\" - - arn:aws:elasticloadbalancing:us-east-2:123456789012:targetgroup/my-targets/73e2d6bc24d8a067 \"Root=1-58337364-23a8c76965a2ef7629b185e3\" \"-\" \"-\" 0 2018-11-30T22:22:48.364000Z \"forward\" \"-\" \"-\"", "http 2018-11-30T22:23:00.186641Z app/my-loadbalancer/50dc6c495c0c9188 192.168.131.39:2817 - 0.000 0.001 0.000 502 - 34 366 \"GET http://www.example.com:80/ HTTP/1.1\" \"curl/7.46.0\" - - arn:aws:elasticloadbalancing:us-east-2:123456789012:targetgroup/my-targets/73e2d6bc24d8a067 \"Root=1-58337364-23a8c76965a2ef7629b185e3\" \"-\" \"-\" 0 2018-11-30T22:22:48.364000Z \"forward\" \"-\" \"LambdaInvalidResponse\"" ]
動作確認
サンプルログをFirehoseに投入するLambdaを実行、Firehose、S3、Athena の 確認を行いました。
Firehose
CloudWatchのメトリック、「Incoming (Bytes / Records)」「SucceedConversion (Bytes /Records)」より正常処理状況を確認します。
「FailedConversion」のメトリックに値が存在する場合、ログファイル(Firehoseのエラー出力先に生成)よりエラー原因を調査します。
S3
FirehoseがPaquet形式でS3に出力したファイルを、S3 Selectを利用して確認します。
Athena
Firehose 出力先としたGlueのデータベースとテーブルを指定し「パーティションのロード」(MSCK REPAIR TABLE alblog;) を行います。
パーティションのロード後、SQLを実行して テーブルに登録されたサンプルログが確認可能となります。
Athena比較
1日、24時間分のALBのアクセスログ、オリジナルの列志向のCSV(スペース区切り)と、 Firehose で 列指向のparquet形式に変換したデータを用意。 ログのタイムスタンプが10時台のログの件数と、転送容量の合計値を求めるクエリをAthenaで実行しました。
CSV のスキャンサイズ55MBに対し、parquet形式では カラムの限定、パーティション利用によりスキャン容量が削減され、 Athena費用(スキャン1TBあたり5ドル)を抑制できる事が確認出来ました。
保存形式 | Where句 | 実行時間 | スキャンしたデータ: | スキャン容量比較(CSV比) | ログ件数 |
---|---|---|---|---|---|
CSV | time LIKE '2019-09-15T10:%' | 2.73 秒 | 55 MB | 100% | 33928 |
Parquet | dt = from_iso8601_date('2019-09-15') and time LIKE '2019-09-15T10:%' | 3.74 秒 | 6.12 MB | 11.1% | 33928 |
Parquet | dt = from_iso8601_date('2019-09-15') and hour in (9,10,11) and time LIKE '2019-09-15T10:%' | 2.85 秒 | 1017.16 KB | 1.8% | 33928 |
Parquet | dt = from_iso8601_date('2019-09-15') and hour in (9,10) and time LIKE '2019-09-15T10:%' | 2.75 秒, | 683.68 KB | 1.2% | 31325 |
Parquet | dt = from_iso8601_date('2019-09-15') and hour in (10,11) and time LIKE '2019-09-15T10:%' | 2.48 秒 | 650.29 KB | 1.2% | 31203 |
Parquet | dt = from_iso8601_date('2019-09-15') and hour in (10) and time LIKE '2019-09-15T10:%' | 2.53 秒 | 316.82 KB | 0.6% | 28600 |
Parquet | dt = from_iso8601_date('2019-09-15') and hour in (10) | 2.5 秒 | 63.23 KB | 0.1% | 28600 |
SQL
CSV
- 1日分のテーブル作成
CREATE EXTERNAL TABLE IF NOT EXISTS alblogcsv( type string, time string, elb string, client_ip string, client_port int, target_ip string, target_port int, request_processing_time double, target_processing_time double, response_processing_time double, elb_status_code string, target_status_code string, received_bytes bigint, sent_bytes bigint, request_verb string, request_url string, request_proto string, user_agent string, ssl_cipher string, ssl_protocol string, target_group_arn string, trace_id string, domain_name string, chosen_cert_arn string, matched_rule_priority string, request_creation_time string, actions_executed string, redirect_url string, lambda_error_reason string, new_field string ) ROW FORMAT SERDE 'org.apache.hadoop.hive.serde2.RegexSerDe' WITH SERDEPROPERTIES( 'serialization.format' = '1', 'input.regex' = '([^ ]*) ([^ ]*) ([^ ]*) ([^ ]*):([0-9]*) ([^ ]*)[:-]([0-9]*) ([-.0-9]*) ([-.0-9]*) ([-.0-9]*) (|[-0-9]*) (-|[-0-9]*) ([-0-9]*) ([-0-9]*) \"([^ ]*) ([^ ]*) (- |[^ ]*)\" \"([^\"]*)\" ([A-Z0-9-]+) ([A-Za-z0-9.-]*) ([^ ]*) \"([^\"]*)\" \"([^\"]*)\" \"([^\"]*)\" ([-.0-9]*) ([^ ]*) \"([^\"]*)\" \"([^\"]*)\"($| \"[^ ]*\")(.*)' ) LOCATION 's3://xxx/AWSLogs/000000000000/elasticloadbalancing/ap-northeast-1/2019/09/15/'
- 10時台のログ集計
SELECT count(1) AS count_rec , sum(sent_bytes) AS sum_sent_bytes FROM "alblogcsv" WHERE time LIKE '2019-09-15T10:%'
parquet
- 時間(hour)のパーティションとして10時台と前後1時間を指定し、10台のログを集計
SELECT count(1) AS count_rec , sum(sent_bytes) AS sum_sent_bytes FROM "alblog" WHERE dt = from_iso8601_date('2019-09-15') and hour in (9,10,11) and time LIKE '2019-09-15T10:%'
コスト
圧縮状態で1GBのALB アクセスログ 、東京リージョンのLambdaとFirehose を用いて parquet形式に変換するAWS利用費は 0.63$となる 計算となりました。
- ALBアクセスログの容量(gzip圧縮済) 1GB
- Firehose取り込みデータ量10GB (圧縮展開とJSON変換による増量)
項目 | 容量 | 金額(USD) |
---|---|---|
Lambda実行時間 (128MB) | 2.5万秒 | 0.052 |
Lambdaリクエスト | 1000リクエスト | 0.00002 |
Firehoseデータ形式の変換 | 10GB | 0.22 |
Firehose取り込みデータ | 10GB | 0.36 |
計 | - | 0.63202 |
まとめ
ALBのアクセスログ の ETL処理 を サーバレスに実現する事ができました。
直近(10分前〜)に発生した ALBのアクセスログ、ステータスコード、IPアドレス といった項目別の集計や抽出を 頻繁に実施する必要がある場合には、Firehoseと Lambda を利用した ニアリアルタイムな parquet形式への変換が効果的です。
S3 のイベントをトリガーに Firehoseへの登録まで実施するサンプルと、利用例については次の記事で紹介予定です。
尚、日時や毎時のバッチで parquet形式に変換を行う場合、Firehose より低コストで利用できる、AthenaのCTASや Glue の Python Shell もお試し下さい。
テンプレート全文
今回紹介した、Lambda、Firehose、Glueを展開する CloudFormation テンプレートです。