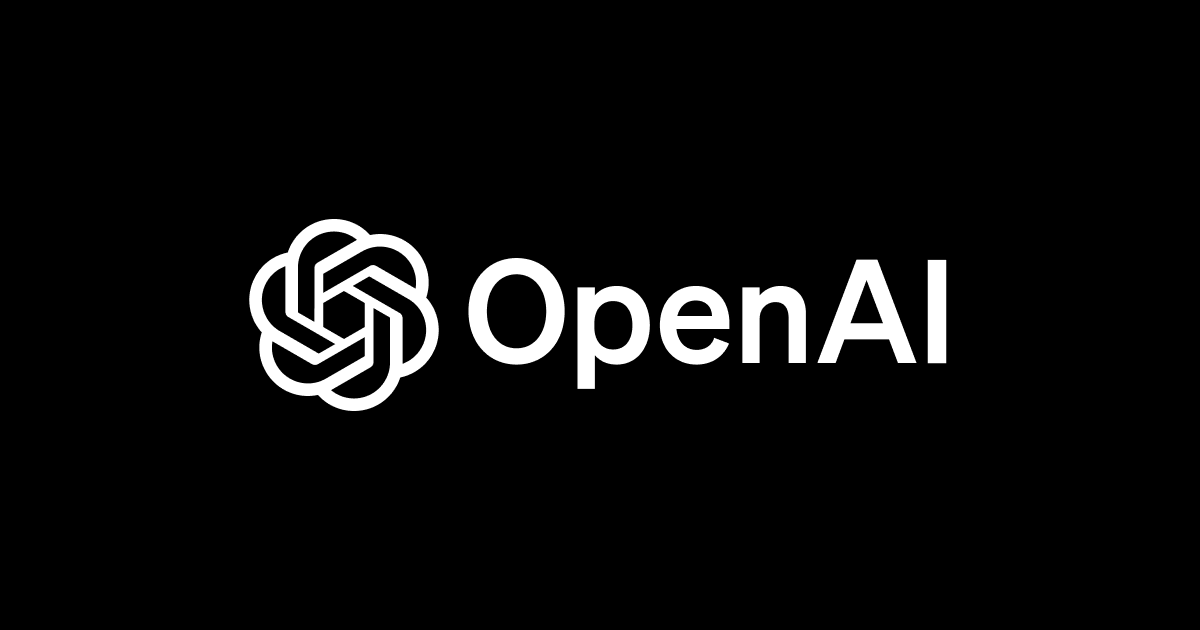
OpenAI’s Text Completion API
Introduction
This blog aims to Call OpenAI's Text Completion API. It is a potential tool for producing text using explicit language models which are powered by OpenAI's GPT (Generative Pre-trained Transformer) models.
Using Completion API we can produce text in many different formats like natural language text, code, SQL queries, etc. The API operates by accepting a portion of a prompt as input and producing the remainder of the prompt as output.
Before we proceed, note that this blog is a continuation blog. The parameters and tables mentioned here are described in detail in the previous blogs, please refer. For reference, I will just add relevant heads-up here.
combine_prompts
- is a function to combine table definition and user query input.df
- dataframe of CSV file. It's some SALES-related data with few columns.nlp_text
- in the user query input in natural language.SALES
- df is modified into a SQL table named SALES.temp_db
- is the SQLite engine that's created.
OpenAI Completion API call
openai.Completion.create
is a method provided by the OpenAI Python client library that allows you to make requests to the OpenAI Completion API. Here's the basic syntax for using openai.Completion.create:
response = openai.Completion.create( model = 'text-davinci-003', prompt = combine_prompts(df,nlp_text), temperature = 0, max_tokens = 150, top_p = 1.0, frequency_penalty = 0, presence_penalty = 0, stop= ['#', ';'] )
To understand better let's explore more about the parameters:
model/engine
: The ID of the GPT model to use for generating completions. You can find a list of available models on the OpenAI website. The default value is "davinci", but we are using one of the powerful models calledtext-davinci-003
.prompt
: The text prompt to use as input for generating completions. This can be a string or a list of strings.temperature
: Controls the level of randomness in the generated completions. A higher temperature value will result in more diverse and unpredictable completions, while a lower value will result in more conservative and predictable completions. The default value is 0.5, but it can range between 0 to 1.max_tokens
: The maximum number of tokens (words or subwords) to generate in each completion. The default value is 16.Top P
: an alternative totemperature
, also known as nucleus sampling. Controls the diversity of the generated text. The model considers the results of the tokens with top_p probability mass. 0.1% means only tokens comprising the top 10% probability mass are considered.n
: The number of completions to generate per prompt. The default value is 1. Ideallyn
can be given more than 1 for exploring different responses, but make suretemperature
> 0 or we might end up getting the same results.Frequency_penalty
: ranges between -2.0 to + 2.0. Positive values penalize new tokens based on their existing frequency in the text. (to break repetitive context cycles)Presence_penalty
: ranges between -2.0 to + 2.0. Positive values penalize new tokens based on whether they appear in the text on their own. Self-analysis to reduce repetitive tasks and include new topics.stop
: A string or list of strings that, when encountered in a generated completion, indicates that the completion is complete and should be returned. By default, this is set to None, which means that the model will generate completions until the max_tokens limit is reached.
In fact, not all parameters are mandatory, there are a few most commonly used parameters like model
, prompt
, max_tokens
, temperature
etc. Try and explore as per the requirements. To try and understand more about parameters you can use OpenAI's playground
Now let's call the API, with above-defined parameters and user query as "return the sum of SALES per POSTALCODE".
To see the SQL Query, extract the text
from response
as follows
response['choices'][0]['text']
Do you see the magic? It's a SQL query…!
We see the query is missing select, so let's concat SELECT
to the query as follows.
def handle_response(response): query = response['choices'][0]['text'] if query.startswith(' '): query = 'SELECT'+query return query
Let's try the query on our SQL table.
with temp_db.connect() as conn: result = conn.execute(text(handle_response(response)))
and the results...
Finally, We could convert a natural language query into a fully working SQL query.
Happy learning, Thank you.