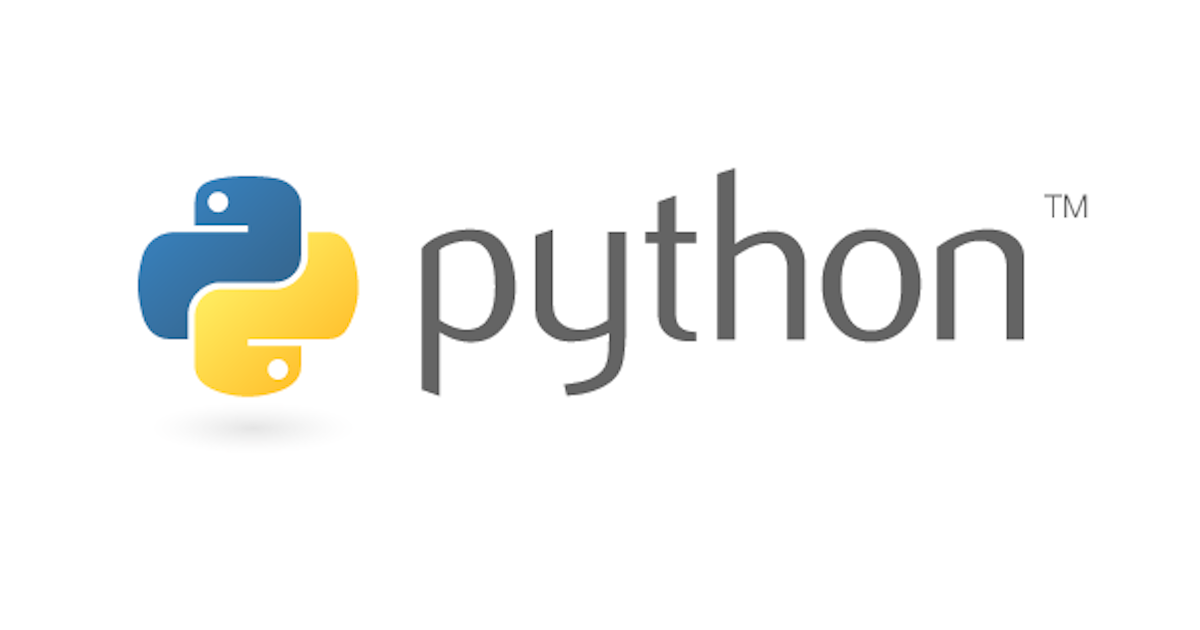
scikit-learnのpipelineモジュールで機械学習パイプラインを作る
データアナリティクス事業本部の鈴木です。
今回はscikit-learnのpipelineモジュールをご紹介します。
pipelineモジュールとは
sklearn.pipelineはtransformerとestimatorを組み合わせて、機械学習パイプラインを構築するためのAPIです。
6.1. Pipelines and composite estimators — scikit-learn documentation
メリットとして以下の3つが紹介されています。
- いくつかの前処理を実行しているような場合にも、fitやpredictをパイプラインに対して1度だけ呼べば良く、便利になる。
-
パイプラインに対してgrid searchする際、パラメータを1度に指定でき、利便性が高い。fitを呼んだ後の変換器をキャッシュでき、性能向上が期待できる場合がある。
-
transformerとestimatorに同じデータが使われるため、CVの際にデータがリークする不具合を防ぐことができる。
まず今回は、簡単な例で使い方を確認してみましょう。
なお、transformerとestimatorはそれぞれ、scikit-learnの主なオブジェクトの一つです。transformerは変換、estimatorは学習を担います。
Developing scikit-learn estimators — scikit-learn documentation
やってみる
検証した環境
- コンテナ:jupyter/datascience-notebook
- scikit-learn:0.24.2
データの準備
今回は、scikit-learnのdatasetsにある、wine datasetを利用します。
多クラス分類を目的としたデータセットで、178サンプルが格納されています。target
にはclass_0・class_1・class_2の3種類があります。
以下のようにして、PandasのDataFrameに変換しておきます。
from sklearn.datasets import load_wine data = load_wine() X = pd.DataFrame(data["data"], columns=data["feature_names"]) y = pd.DataFrame(data["target"], columns=["target"]) X.head()
Xおよびyに欠損値はありません。
X.isnull().sum() ## alcohol 0 ## malic_acid 0 ## ash 0 ## alcalinity_of_ash 0 ## magnesium 0 ## total_phenols 0 ## flavanoids 0 ## nonflavanoid_phenols 0 ## proanthocyanins 0 ## color_intensity 0 ## hue 0 ## od280/od315_of_diluted_wines 0 ## proline 0 ## dtype: int64 y.isnull().sum() ## target 0 ## dtype: int64
とりあえず、train_test_splitで訓練用とテスト用にデータを分けておきます。
from sklearn.model_selection import train_test_split X_train, X_test, y_train, y_test = train_test_split(X, y, random_state=42)
パイプラインを構築する
wine datasetの特徴量が全て量的変数なので、全特徴をStandardScalerで標準化した後、HistGradientBoostingClassifierでクラスを推定します。
from sklearn.pipeline import Pipeline from sklearn.preprocessing import StandardScaler from sklearn.experimental import enable_hist_gradient_boosting from sklearn.ensemble import HistGradientBoostingClassifier pipe = Pipeline(steps=[("standerd_scaler", StandardScaler()), ("Classifier", HistGradientBoostingClassifier())])
Pipelineのコンストラクタのstep引数に、名前とtransformerもしくはestimatorのタプルのリストを渡します。
make_pipeline(Pipelineのコンストラクタの省略形)を使うことで、各ステップに名前を付けずにパイプラインを構築することも可能です。
from sklearn.pipeline import make_pipeline make_pipeline(StandardScaler(),HistGradientBoostingClassifier()) ## Pipeline(steps=[('standerd_scaler', StandardScaler()), ## ('Classifier', HistGradientBoostingClassifier())])
想定どおりにできているかどうかは、以下のように確認できます。
pipe ## Pipeline(steps=[('standerd_scaler', StandardScaler()), ## ('Classifier', HistGradientBoostingClassifier())])
set_configの設定を変えることで、文字ではなく、ダイアグラムで確認することもできます。
from sklearn import set_config set_config(display='diagram') pipe
学習と推論をしてみる
パイプラインを使って、学習と推論をしてみます。
パイプラインのfit
、predict
やscore
などを呼ぶことで、パイプラインに登録した前処理が行われた後、estimatorの該当するAPIが呼ばれます。厳密にはestimatorはfit
を実装しているだけのようですが、今回パイプラインに組み込んだHistGradientBoostingClassifierはpredict
やscore
も実装しているので、問題なく実行できます。
# 訓練 pipe.fit(X_train, y_train.values.ravel()) # 推論 pipe.predict(X_test) # モデルのscoreで評価する pipe.score(X_test, y_test) ## 0.9777777777777777
また、クロスバリデーションも、sklearn.model_selectionモジュールのcross_val_scoreにそのままパイプラインを渡すことで可能です。
from sklearn.model_selection import cross_val_score scores = cross_val_score(pipe, X_train, y_train.values.ravel(), cv=5, scoring='accuracy')
最後に
pipelineモジュールを使うことでtransformerとestimatorによる一連の処理を簡潔に記載することができました。
今回は全てのカラムに対して一連の前処理を行うケースを紹介しました。実際には各特徴に対してそれぞれ異なる前処理をしたい場合など、もう少しパイプラインは複雑になります。そのようなケースは別の記事でご紹介します。