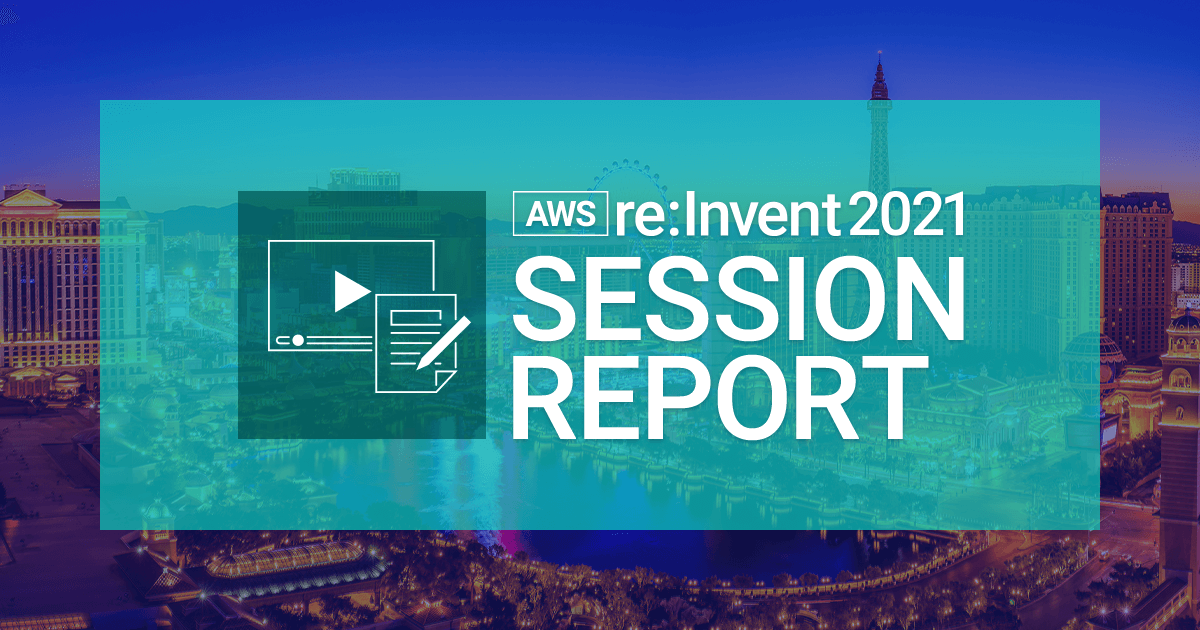
【現地セッションレポート】 {New Launch} Introducing AWS Trainium-based Amazon EC2 Trn1 instances #CMP334#reinvent
新規事業統括部の山本です。
AWS re:Invent 2021に参加し現地のセッションを受けてきたので、内容をレポートします。
今回は、キーノートで発表された新機能の1つである、EC2 Trn1インスタンスに関するbreakoutセッションです。
発表内容まとめ
内容をまとめると以下のようでした。
- Trn1は分散学習に適したHW性能・設計されているので、学習を速く回せる
- 速度に関する数値的な話はなかった
概要
セッションカタログの内容は以下の通りです。
Title: {New Launch} Introducing AWS Trainium-based Amazon EC2 Trn1 instances
Code: CMP334
Session type: Breakout Session
Topics: Compute
Session level: 300 - Advanced
Introducing the new Amazon EC2 Trn1 instances featuring AWS Trainium, the second ML chip built by AWS that is optimized for ML training. Attend this session for a first look at how Trn1 instances deliver the best price performance for training deep learning models in the cloud for natural language processing, object detection, image recognition, and other use cases. Dive deep into how AWS Trainium was architected to deliver the fastest model training in Amazon EC2.
発表内容
AI/MLは広く使われている、大企業・アカデミックにとどまらない
AI/MLのトレンド
- モデルが複雑になり、サイズの大きくなっている ^ 学習にかかる時間が数日レベルから数週間レベルになっている
ML/DL関連の革新
Trainiumを発表
Trn1
- 高性能なHW
Trn1の性能
- BW=BandWidth
Trainiumのアーキテクチャ
cFP8という新しい数値タイプ
- 速度と計算精度のちょうど良いところ
- 数値精度とスケールを調整可能
学習時間が長くなっているから、スケールアウト(=分散学習?)が必須
分散学習の話
- 3つ方法がある
データ並列化
- それぞれのワーカにおいて、別のバッチで計算を実行
- 欠点(=必要なHW要件):大きなメモリサイズが必要
パイプライン並列化
- 各ワーカに対して、モデルのレーヤごとに配置し、各データを並列に順次処理する
- backwardがあるため、通常の処理だと無駄が多いが、各データを並列に実行するので、idle時間が少ない
- 欠点:大きなメモリサイズが必要、計算・通信が同時に発生する
Tensor並列化
- レイヤーをまたいでモデルを分割
Recap:並列化まとめ
- 上の3つをまとめると、効率的な並列化には4点が必要
Trn1はこうした並列化に適したハードウェア性能・設計をしている
- (下画像は4点目のスライド、他は追いつけず撮影できませんでした)
SDKで並列化を指定できる